One of the major challenges in building automated supervised image classification systems is the amount of training data that needs to be correctly identified. In such systems, hundreds or thousands of correctly labeled images need to be presented to the system since more labeled data will result in a system more effective than one where just a few data is presented to the classifier (see "Machine learning leverages image classification techniques," Vision Systems Design, February 2015). However, generating such labeled data is often a manual and laborious process since similar objects within images may be rotated, scaled or translated in numerous ways.
"In the development of an obstacle avoidance system for an automotive manufacturer," says Dr. Daniel Kondermann, President of Pallas Ludens (Mannheim, Germany; www.pallas-ludens.com), "hundreds of thousands of images such as automobiles, pedestrians, trees, and other obstacles need to be properly identified at multiple scales." Similarly, to build such a system to identify brain tumors in MRI images, a physician must isolate the relevant pixel regions in multiple images. Although global segmentation algorithms may be used to perform this task," says Kondermann, the results of these algorithms may be ambiguous and not as accurate as those performed by an expensive human operator."
Realizing this, Pallas Ludens has developed an elegant way to solve the task of rapid image classification development. Instead of requiring a single trained professional to identify multiple images or objects within images, the company has leveraged the power of crowd sourcing to complete the task (Figure 1).
"Companies in the medical, automotive and entertainment industries require classification systems that must be as accurate as possible," says Kondermann. When approached by such companies, Pallas Ludens recruits thousands of Internet users through both on-line work marketplaces and computer game users to perform the task of image identification. Using Amazon's Mechanical Turk (www.mturk.com), for example, users are presented with examples of correctly classified images and then asked to label unknown images based on these examples.
In the case of an MRI image, for example, this may involve drawing a contour around a region of interest. In the case of an obstacle avoidance system, this may require highlighting a pedestrian. Performing such tasks, users of Amazon's Mechanical Turk can earn between $3-6/hour, (see "Conducting behavioral research on Amazon" by Winter Mason and Siddharth Suri; http://bit.ly/1wzhKz1).
To gain even more users, Pallas Ludens has teamed with Bigpoint (San Francisco, CA; www.bigpoint.com), a maker of online games such as Dark Orbit and Rising Cities. Here, the user can earn "virtual currency" when playing the game by labeling images provided within the game by Pallas Ludens.
"Europeans often do not like parting with their credit card information to buy such virtual currency. However, they are willing to work of specific task while playing these games to earn such currency," says Kondermann. In this model, Bigpoint is paid by Pallas Ludens for access to its large installed user base. Once such images have been labeled, they are combined on Pallas Ludens' server and presented to the company's client.
Already a large automobile manufacturer has used the company's software in the development of an obstacle avoidance system. "After thousands of labeled images are delivered," says Kondermann, "a classification algorithm is embedded in the automobile's navigation system to identify images from streaming video captured by multiple cameras on the automobile. If an image is deemed to be too close to the automobile, a warning signal is then issued to the driver."
While automobile companies may use their own classification algorithm for this task, Pallas Ludens is developing its own classifier based on a random forests algorithm that, Kondermann says, is similar to other learning methods such as deep neural networks.
With such developments, the time to develop image classification tasks can be reduced dramatically. With the number of users now in place, Pallas Ludens claims performance metrics for image labeling as high as six man years/day.
According to Kondermann, the company is currently seek additional funding to develop additional user interfaces, expand its web network and develop more sophisticated image classifiers.
About the Author
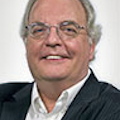
Andy Wilson
Founding Editor
Founding editor of Vision Systems Design. Industry authority and author of thousands of technical articles on image processing, machine vision, and computer science.
B.Sc., Warwick University
Tel: 603-891-9115
Fax: 603-891-9297