Novel stitching software powers high-resolution video capture system
Unveiled at CES 2020, a system from RayShaper (Crans-Montana, Switzerland; www.rayshaper.ch) combines multiple cameras and proprietary software for an immersive, high-resolution video system aimed at changing the way people stream unscripted events or applications like sports, concerts, or surveillance.
Co-founder Touradj Ebrahimi, who led the groups that developed the JPEG 2000 and MPEG-4 standards, explains that the physical system resembles a beehive (Figure 1) and comprises a minimum of three commercially-available, 8 MPixel CMOS cameras capable of 120 fps each. The setup is also entirely customizable based on customer requirements.
“Customers can choose the number of cameras needed based on the required picture quality an application requires, and the way the sensors should be configured” he says. “If more pixels or higher dynamic range are necessary, then more cameras can be built onto the system, like with Legos.
He adds, “Behind this, however, is the patented software which combines the different outputs for every sensor to create a high-quality image or video. This is the secret sauce.”
Rayshaper’s software performs image stitching, tracking, and analysis operations. Stitching allows for real-time creation of a single ultra-high-resolution, high-dynamic range and high-quality image from multiple sub-images captured from a same scene. In its most typical configuration, all sensors in the camera point to the same direction, with some lenses set up to a wider and some to a narrower viewing angle, resulting in sub-images representing different areas of the scene but in different resolutions, while overlapping.
Furthermore, the software performs the important function of synchronization. When the camera operates in video mode in high frame rates, they must be synchronized so that the software stitches sub-images captured at exactly the right time. Both the stitching and synchronization tools in the software are patented technologies that sit at the core of the system. Tracking of objects and video analytics can be performed on the ultra-high-resolution video, either for interfacing with other software and data bases, or for personalization of the content, for instance, in automatic creation of personalized highlights from ultra-high-resolution clips of a Formula 1 car racing.
“Many stitching solutions today are either in the camera in real time and low quality, or done offline which takes quite a while,” says Ebrahimi. “Rayshaper’s system combines the quality of offline solutions for stitching, with real-time feature desired in live application scenarios.”
The software’s stitching tool is based on optical flow, meaning it extracts information from the pattern of visual motion between an observer and a scene. The tool also uses deep learning algorithms to learn from temporal information to improve stitching results, explains Gene Wen, CEO. Eventually the software learns gradually how images should be stitched through deep learning. Due to various factors, however, stitching results may vary with time. For example, in shooting outside in very cold or hot weather.
“Slight changes to the lens may exist due to temperature, so the deep learning algorithm slowly learns the parameters on the fly to adjust stitching results for optimal results,” he says. “The design also allows developers to deploy different lens configurations.”
“For example,” he says, “four sensors may have the same lens but there may be slight differences in their angles. When this occurs, our algorithm learns to adjust to such tolerances over time for stitching.”
In this system, deep learning also assists in tracking people or objects in scenarios like sports or surveillance and in situations where fog, smoke, or low lighting may impact image or video quality. Since fixed parameters cannot be used due to these conditions, the algorithms adapt to the operating conditions of the application to adjust for color or brightness correction, stitching, low-light enhancements, and defogging. The algorithms, according to Wen, learn and adjust to these conditions over time by comparing information from different lenses, for example.
In a train station surveillance application, for instance, artifacts like pollution and smoke entered the frame of the video system and must be corrected. The deep learning algorithms are built into the stitching process and make these adjustments to improve the end user’s results.
“If one were to put a polarizer on one lens, for example, the algorithm will detect the need for correction and make those adjustments,” says Wen.
In addition to stitching, analysis, and tracking, RayShaper’s software renders images and video at what the company calls gigapixel resolution. While not actually a billion pixels, the combination of multiple sensors and proprietary software enables a similar capability. According to the Shannon-Nyquist theorem, if a limited bandwidth continuous signal is sampled above the Nyquist sampling rate, faithfully reconstructing the original signal without loss of information is possible.
With a sine signal of frequency f, sampling at 2f, 3f, 4f will produce a corresponding number of samples per period. In the case of RayShaper’s software, this amounts to 1/f. Since reconstructing the sine signal 100% from sampling at 2f (2 samples/period) is possible, it is also possible to produce samples for sampling at 3f, 4f, and so on, as if sampling was done at these frequencies. Actual resolution is the sampling resolution, which must be above the theoretic lower limit, which in this example is 2f, explains Wen.
“For example, it is possible to take a picture of a perfectly flat and white piece of paper at VGA resolution and produce a 4K image without losing or fabricating any information,” he says. “In RayShaper’s case, if we use uniform distribution of resolution both temporally and spatially, to provide the details for the close-ups, we would need over 1 billion pixels uniformly distributed. Instead, the algorithm allows us to have the same capability with fewer cameras.
A typical setup consists of the camera system connected to a PC for either real-time, offline, or post processing, for tasks like noise reduction or color correction. Depending on the application and the number of sensors, graphics processing units (GPU) may be required as well.
The system’s multi-sensor setup also enables light field acquisition, which Ebrahimi says enables users to focus in on different planes on different objects from different distances. In traditional image acquisition, one must decide which object or distance should be in focus, and anything too far or too close becomes out of focus. For example, if a surveillance system follows a car with the car in focus, but in a window or another car farther away, the system might be able to identify a driver or a read a license plate.
“Two ways exist to acquire light field images, the first of which is a light field camera using micro lenses such as the one made by Lytro,” says Ebrahimi. “The other way is using an array of sensors in a matrix configuration, and both approaches receive and send light to the sensor from several directions. This enables the end user to go back and put any object in the field of view into focus.”
In terms of applications, Rayshaper’s product has been used to capture video for live music, where it may be difficult for the camera operator to zoom in on the right musician at the right time, or for analyzing and tracking different players in sports (Figure 2).
“Because of the ultra-high-resolution of the compound acquisition system, a producer does not have to decide which player to track in a hockey game, for example,” says Ebrahimi. “Instead, it automatically tracks all the players and helps the producer to follow all players as if there were five camera operators following the action.”
“Same for live music,” he adds,” where a lot of things can be happening at once on the stage. Sometimes the video should show a guitarist, trumpet player, and so on, and the software can capture the full band quite nicely.”
Looking toward future uses, Wen suggests that the setup could combine visible camera information with infrared or hyperspectral data, for applications such as precision agriculture, surveillance, or even viewing a concert at night.
About the Author
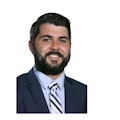
James Carroll
Former VSD Editor James Carroll joined the team 2013. Carroll covered machine vision and imaging from numerous angles, including application stories, industry news, market updates, and new products. In addition to writing and editing articles, Carroll managed the Innovators Awards program and webcasts.