Challenges and Emerging Applications for Deep Learning/AI
At this stage, few in imaging are unaware that deep learning and artificial intelligence (AI) are benefitting vision/imaging applications. Much like any new technology, there’s a lot of talk about it when it first arrives. Some decide to wait for Gen 2 of Gen 3 of a technology before jumping in, while others are early adopters. For deep learning/AI, challenges to implementation remain, although new applications for it continue to emerge.
Challenges
Lack of AI experience continues to be a challenge for those looking to deploy deep learning/AI solutions for their customers as well as the dataset and expense of the project. Massimiliano Versace, CEO and Co-Founder of Neurala (Boston, MA, USA; www.neurala.com), says “We often find that the biggest challenge is usually one of a lack of AI expertise, the overall expense of the project, or a lack of quality data to be able to train a model, in particular a lack of images of defective parts. Today’s manufacturers require flexible, cost-effective, easy-to-deploy solutions.”
Brandon Hunt, Product Manager, Teledyne (Waterloo, Ontario, Canada; www.teledynedalsa.com), concurs that lack of experience continues to challenge implementers. “By far the biggest challenge is education/training or a lack of expertise in deep learning/AI,” he says. “AI is still emerging in machine vision and most companies lack the proper knowledge to effectively identify and implement AI-enabled vision systems. Some are saying it is similar to the early days of machine vision.”
While lack of AI experience is a challenge, addressing it is also a challenge, as Robert-Alexander Windberger, AI Specialist, IDS Imaging Development Systems (Obersulm, German; www.ids-imaging.com) comments that the challenge is, “Addressing the shortage of skilled vision and AI experts, by removing the requirement for programming skills and AI background knowledge, in order to scale up the technology.” He also adds that other challenges include reducing the high costs associated with data management and storage. He continues, “We focus our efforts not only on data, but also on the people who are developing AI vision systems. Our goal is to equip them with the right tools, so that they can work efficiently towards their AI solution and enable them to understand and control each step along the AI process.”
This can also be explained as ease of installation, and Eli Davis, Lead Engineer at DeepView (Mt. Clemens, MI, USA; www.deepviewai.com) says, “As deep learning matures, the setup process is being streamlined. In the last several years the biggest challenge to deploying deep learning was gathering enough training images from customer parts. Applications that required 400 training examples in 2021 are being deployed with 40 training examples in 2023. This is achieved by deploying deep learning models with more visual intelligence built-in than ever before.”
Along with experience with AI, another challenge involves the data required to build a model. Luca Verre, CEO and co-founder of Prophesee (Paris, France; www.prophesee.ai), says, “Any AI application is going to require a significant amount of data processing performance. This can be addressed relatively easily in use cases that have access to large amounts of computing and power resources. The volume of data becomes a more challenging issue when we begin to look at applications at the edge or in mobile applications. And, these happen to be areas where vision is a much more critical feature. In operating scenarios such as a remote camera, in a car, in a wearable device such as an AR headset, or in a mobile device AI-enabled vision needs to be implemented in a much efficient way so that the necessary data can be processed in a practical, useful and timely way. And of course, power efficiency is critical as well. As a result, vision application developers are looking more and more at approaches such as event-based vision that capture and process only data in scenes that change. Event-based vision can address the data processing and power challenges of implementing AI vision, and also offer a solution that works more efficiently in challenging lighting conditions.”
Martin Bufi, AI Technology Manager at Musashi AI (Ontario, Canada; www.musashiai.com), adds, “The biggest challenge for implementing deep learning/AI is the availability of large amounts of high-quality data and computational resources. Deep learning algorithms require a vast amount of data to train and validate their models, and they can be computationally intensive. Additionally, it can be challenging to design deep learning models that generalize well to new, unseen data, and to interpret the internal workings of these models.”
Yoav Taieb, co-founder and CTO, Visionary.ai (Jerusalem, Israel; www.visionary.ai), also cites datasets. “The biggest challenge for implementing deep learning today is in obtaining large-scale datasets, which are both clean and applicable,” he says. “Datasets frequently contain ‘dirty’ data. For example, if we look at image classification datasets, we frequently will encounter data that are not labeled accurately (for example, a dog labeled as a cat) or that haven’t been defined well enough.” He adds that equally challenging in a dataset is the need for the data distribution to reflect real-world distribution as much as possible. “If we use dogs as an example, sausage dogs might only represent a tiny percentage of all dogs in real-world distribution,” he says. “But if 50% of the dogs in the dataset are sausage dogs, then the training won’t be accurate, and neural networks will struggle to make accurate classifications.”
Pierantonio Boriero, Senior Manager, Product Management – Software, Machine Vision and Imaging, Zebra Technologies Corporation (Lincolnshire, IL, USA; www.zebra.com), adds, “Integrating deep learning (DL) models into existing systems and workflows can be challenging, and deploying models at scale can be complex and resource-intensive. The preparation involved in developing and deploying such a system requires collecting large, high-quality image datasets then appropriately labelling these images before the model training and refinement begins. The process can be quite iterative and time consuming.”
“Nowadays, academic experts say that improving data is more advantageous for improving the performance of models,” says Hongsuk Lee, CEO, Neurocle, Inc. (Seoul, The Republic of Korea; www.neuro-cle.com). “To practice such a data-centric view, massive amount of high-quality data for model training and personnel with high understanding of the data are required. However, this is a big challenge for manufacturer since it is hard to acquire massive amount of data from the sites where the incidence of defect occurring is very low. In addition, industrial engineers with a high understanding of data usually do not have professional knowledge of deep learning, which inevitably results in the effort and cost of hiring deep learning engineers or outsourcing from outside.”
Emerging Applications
Deep Learning/AI is one technology that has pushed machine vision farther away from the factory floor and allowed it to be deployed in more non-factory settings. As the challenges above are addressed, new applications are uncovered.
For example, according to Versace, “Most of the applications we see today are using AI to inspect the quality of parts or products. We are now beginning to see applications where AI is monitoring processes for correctness or completion. For example, has assembly been completed in the correct sequence? We’re also seeing AI applied beyond visual applications into sensor monitoring within machines to predict failures and malfunctions.”
Other areas of growth include manufacturing, electronics, semiconductor, automotive, and food and recycling industries, although Hunt says AI is still largely underused in machine vision. “The applications include anywhere there are high amounts of variation in lighting/ defects—the food industry is perfect for this as nature contains this naturally,” he says. “Some top applications are defect inspection for areas like automated optical inspection (AOI) of PCBs, surface inspection of materials (sheet metal, aluminum can, fabric), and battery inspection. We are seeing more growth and investment in the electric vehicle industry, so battery inspection will be an increasing trend here.”
Regarding battery inspection, Lee states, “As the electric vehicle market expands, the global secondary battery industry continues to grow significantly. The world’s major secondary battery industries are pushing for a large-scale expansion in production that scales more than three times that of 2020 capa by 2025. In addition, it is expected that the supply of secondary batteries could be insufficient from 2023 due to the rapid growth of the electric vehicle market. Reliable quality inspections are essential in the sector, which is used in electric vehicles and is directly related to human safety. However, the quality test of secondary battery poses more issues than any other industry because the standard for defect test has not yet been standardized.”
At Prophesee, Verre says, “The most interesting area we are seeing is the continued movement of intelligent sensing to the edge. This mandates a much more efficient and flexible approach to implementing AI and ML for all sensing applications, and especially vision. As a result, AI-enabled machine vision systems must now operate at new levels of efficiency. By managing the amount of data that needs to be processed, edge and mobile systems can leverage the potential of AI, which reduces power and other operational costs and also meets critical performance KPIs such as response times, scanning times, and dynamic range for low light operation.”
Speaking of the edge, Taieb says, “Perhaps most interestingly, more things will move from the cloud to the edge. I think we’ll see most cameras start using AI chips, and we’ll see more happening on the edge, as it’s easier. Not everything needs to happen in the cloud; the edge is far more efficient. For real-time imaging, the edge is more immediate than in the cloud, which is a significant advantage.”
Christian Eckstein, Product Manager & Business Developer at MVTec Software (Munich, Germany; www.mvtec.com), predicts, “In 2023, we will see AI applications becoming more widespread. Proof of this is that companies have invested in corresponding systems in recent years, and they are now being put into operation one by one. Another point in this direction is the commoditization of many AI methods. Many manufacturers of sensors and smart cameras are releasing easy to use AI products to market. This also lowers the barrier in machine vision to use deep learning applications.”
Another prediction for 2023 and beyond, this time from Gil Abraham, Business Development Director at CEVA’s Artificial Intelligence and Vision business unit (Rockville, MD, USA; www.ceva-dsp.com), is that there will be rapid penetration in the surveillance, autonomous driving, AR/VR SLAM, communication, and healthcare applications and sensing of all types.
Windberger adds, “We expect the market for AI applications to focus on improving and intensifying existing ones rather than expanding into new areas. Despite promising proof of concepts in various fields, many have failed to reach production due to the reasons stated before.” He continues, “Another trend in 2023 will be the continued efforts to increase the transparency of AI systems, which will impact both the predictability of performance and interpretability of decisions. Users will gain a more intuitive understanding of a model's performance and weaknesses through data visualization methods such as confusion matrices."
Finally, Davis says that deep learning broadens the machine vision market to include weld inspection, molded plastics, and metal stampings.
Solutions
There are a variety of products available to address the above trends and challenges. Following is a sample of what is currently on the market.
IDS NXT (Figure 1) is a comprehensive AI-based vision system consisting of intelligent cameras plus software environment that covers the entire process from the creation to the execution of AI vision applications. The software tools make AI-based vision usable for different target groups—even without prior knowledge of artificial intelligence or application programming. In addition, expert tools enable open platform programming, making IDS NXT cameras highly customizable and suitable for a wide range of applications.
Deep Learning Optical Character Recognition (DL-OCR) (Figure 2), from Zebra Technologies, supports manufacturers and warehousing operators who increasingly need fast, accurate, and reliable ready-to-use deep learning solutions for compliance, quality, and presence checks. The industrial-quality DL-OCR tool is an add-on to Zebra Aurora™ software that makes reading text quick and easy. DL-OCR comes with a ready-to-use neural network that is pretrained using thousands of different image samples. Users can create robust OCR applications in just a few simple steps—all without the need for machine vision expertise.
CEVA NeuPro-M™ (Figure 3), Heterogeneous and Secure ML/AI Processor Architecture for Smart Edge Devices is a highly scalable high-performance AI processor architecture with power efficiency of up to 24 Tera Ops Per Second per Watt (TOPS/Watt). NeuPro-M was designed to meet the most stringent safety and quality compliance standards.
MVTec's MERLIC 5.2 (Figure 4) is a logical continuation of the approach taken since MERLIC 5, namely to provide customers with the latest deep learning features that are also easy to use. Global Context Anomaly Detection is now being added to the proven deep learning functions Anomaly Detection and Classification (from MERLIC 5) and Deep OCR (from MERLIC 5.1). As in previous versions, users have access to MVTec's Deep Learning Tool for training the data. The tool is designed for easy labeling and training of data. Both operations are in fact crucial steps for any deep learning application.
Visionary.ai released its real-time image denoiser (Figure 5) which achieves results in low-light. The results of classical denoisers are limited, and so the company developed an AI-based approach, which helps remove more image noise and works in real time. Results showed that the company’s AI ISP denoiser was able to achieve significantly higher precision. The Visionary.ai denoiser achieved precision of 93.03%, compared with 86.96% precision with the classical denoiser and 65.42% with no denoiser. The test focused on an extreme lighting situation where most of the detection problems occur and where most systems fail.
Cendiant™ Inspect (Figure 6) is deep learning-based inspection software developed by Musashi AI as part of a turnkey AI solution for surface inspection of complex parts. In addition to detection and classification of visual defects, the software can be deployed for gauging, process completeness and assembly verification checks. Cendiant Inspect utilizes Active i™ technology where active intelligence is achieved by the AI though a proprietary network architecture of two independent anomaly and segmentation algorithms communicating inspection outputs and optimizing via a designed feedback loop. The result is an AI solution for complex inspection with low data requirements.
Prophesee’s Metavision® EVK4 - HD Evaluation Kit (Figure 7) is an ultra-light and compact evaluation kit built to endure field testing conditions. It contains the Sony IMX636ES HD stacked event-based vision sensor. With the evaluation kit (EVK), anyone can start the evaluation of the stacked event-based vision sensor in an efficient and accurate way to perform full system design, analysis, and experimentation.
Sapera™ Vision Software (Figure 8) from Teledyne DALSA offers AI-enabled machine vision application development for OEM’s and system integrators at scale. The Sapera Vision Software development platform offers a wide range of traditional image processing tools and AI functions, and seamless integration with Teledyne’s hardware portfolio (1D, 2D, 3D). With Astrocyte, Teledyne’s AI training tool, users can rapidly train AI models without any deep learning expertise or code, at a competitive price. Once trained, models are ready for deployment in Sapera Processing (API) or Sherlock (GUI).
Neurala recently added a new feature to its Vision AI platform VIA, called Calibration (Figure 9). The calibration feature allows the user to adjust the deployed AI model in Inspector to account for local environmental variables such as differences in illumination. Calibration allows users to adjust and refine the model locally, in line, based on subtle environmental changes that may have occurred. Calibration simply recalibrates the AI model locally to adjust for these changes so that the model can still perform well without having to retrain the model using data that is representative of the new environment.
Neuro-T 3.2 and Neuro-R 3.2 (Figure 10), Neurocle’s latest release, introduce multimodel utilization and improved data and model management. Users can expect higher work efficiency and stability by predicting the performance of multiple models before actual deployment. New ways to manage data and models increase user convenience and project management efficiency during collaboration. Neuro-T now comes with an Inference Center, a space where users predict and evaluate model performance before deploying a model to the field, allowing users to design projects by visualizing the models and modifying their projects based on inference time predictions.
Companies Mentioned
CEVA
Rockville, MD, USA
www.ceva-dsp.com
DeepView
Rochester, MI, USA
www.deepviewai.com
IDS Imaging Development Systems
Obersulm, Germany
www.ids-imaging.com
Musashi AI
Ontario, Canada
www.musashiai.com
MVTec Software
Munich, Germany
www.mvtec.com
Neurala
Boston, MA, USA
www.neurala.com
Neurocle
Seoul, The Republic of Korea
www.neuro-cle.com
Prophesee
Paris, France
www.prophesee.ai
Teledyne DALSA
Waterloo, Ontario, Canada
www.teledynedalsa.com
Visionary.ai
Jerusalem, Israel
www.visionary.ai
Zebra Technologies
Lincolnshire, IL, USA
www.zebra.com
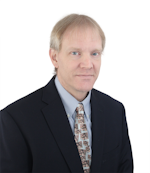
Chris Mc Loone | Editor in Chief
Former Editor in Chief Chris Mc Loone joined the Vision Systems Design team as editor in chief in 2021. Chris has been in B2B media for over 25 years. During his tenure at VSD, he covered machine vision and imaging from numerous angles, including application stories, technology trends, industry news, market updates, and new products.