Interview with hyperspectral imaging and machine vision expert Amrita Sahu
“If a picture is worth a thousand words, a hyperspectral image is worth almost 1,000 pictures,” John Ferguson.
In this interview, data driven modeling and hyperspectral imaging expert Amrita Sahu, Senior Scientist at Altria, talks about some of the challenges she faced while implementing machine vision systems in the clinical and agricultural space, and how she overcame them.
What brought you into this field?
As a young child growing up in India, math and physics were my favorite subjects. I frequently visited the lab where my mother worked as a scientist and clinic where my father was a doctor. Seeing how their work contributed to society was amazing and led to a personal interest in science at school. A passion for science and technology motivated me to apply for graduate schools in the US. During graduate studies at Temple University, I first encountered different machine vision techniques like hyperspectral imaging.
The initial project I worked on was developing a hyperspectral imaging system to detect canine cancer, and being a dog lover, this was quite gratifying. Soon after it became clear that an interdisciplinary field involving physics, optics, mathematics, computer science, and system development was the correct path.
What are some of the challenges you’ve encountered in hyperspectral/multispectral imaging, and how did you overcome them?
Leading an imaging team at Altria, we developed and implemented a hyperspectral imaging based agricultural product grading system. After successful prototyping and testing in a laboratory environment, the system was deployed in an industrial environment. Initial implementation of the grading system utilized a base model of each variety of the crop. However, errors were encountered while applying this to samples from a different crop year. Agricultural crops may vary annually due to difference in external conditions such as weather and soil. Thus, the team began investigating the annual variation of the spectral data and found that the different grades over multiple years showed a shift in time. The team then introduced a mechanism for seasonal calibration where a minimum of six selected samples were utilized to bring the classification model in line with that years’ crop. This procedure is now done for each combination of variety, grade, and growing region. As a result, the hyperspectral system is quite robust year after year.
Another challenge involves degradation of the illumination source over time, which affects image quality. In the setup, a tungsten-halogen light source provided illumination. To detect the degradation of this light without any human intervention, we used a Spectralon standard reference that was reimaged every thirty minutes as a benchmark reference. The procedure enabled the detection of any fluctuations that may be present during a session and not effect end results.
While developing any hyperspectral/multispectral based quality control system, the goal is ensuring the system can be used at multiple locations. However, often hyperspectral imagers have deviation in their operational wavelength from their marked specification. As the team attempted to build a generalized system for different locations, I developed an interpolation technique that maps its recorded data to a set of standard wavelengths. This allows the comparison of data from different installation locations and enables the replacement of a camera without affecting the efficacy of the system.
What are some of the challenges you’ve encountered in machine learning, and how did you overcome them?
Hyperspectral images contain substantial amounts of data and often suffer from the well-known problem of multicollinearity, which poses considerable computational challenges. Spectral feature extraction helps overcome the issue of high dimensionality. While designing hyperspectral systems for quality control, I created a library of spectral endmembers. Depending on the type of crop, five or six endmembers were extracted to represent the spectral characteristic of each target. Sequential Maximum Convex Cone Endmember Model (SMACC) was used as the spectral feature extraction method. Using linear unmixing, abundance values for each type of crop to be used as defining parameters for classification were calculated.
Regression techniques such as Partial Least Squares (PLS) have also helped overcome the problem of multicollinearity in hyperspectral data. PLS explores the linear combination of spectral data and chemical composition. However, the effects of multicollinearity can only be reduced but not completely removed by PLS, but variable selection methods such as successive projections algorithm prove effective by selecting subsets of variables with minimum redundancy and collinearity. This not only improves the predictive power of calibration models, but also simplifies the model by avoiding redundancies and irrelevant variables.
Before system deployment, one should generalize the machine learning model. Overfitting becomes increasingly problematic when model complexity increases. Building a quality control system involved the team collecting spectral data over the course of three years of crop cycle. Spectral data was separated into training, validation, and test set. The validation set tuned the hyperparameters of the model. The model was extensively tested on the test dataset make sure it generalizes and there is no data leakage.
What are some recent challenges you’ve encountered in general machine vision applications, and how did you overcome them?
A challenge encountered while deploying hyperspectral/multispectral imaging for general machine vision applications such as online contaminant detection of food and other agricultural materials, is efficient real-time data processing capabilities to keep up with the factory line speeds. Hyperspectral imaging systems offer massive information about the target, so efficient data processing methods and hardware systems are needed. For this purpose, parallel hardware devices such as graphics processing units (GPU) and field programmable gate arrays (FPGA) work well. Implementation of parallel algorithms on GPUs has significantly improved the real-time classification of hyperspectral images. On the image preprocessing side, both spatial and spectral binning help reduce computational load.
While designing hyperspectral systems for industrial use, physical factors such as temperature, air flow, dust, and prolonged usage can create issues. To combat any complications arising from heat and dust, a metal enclosure was built to house the system. Fans installed on each side of the assembly turn on during the use of the system and maintain a level of negative pressure, ensuring that dust does not settle on the lens of the hyperspectral camera. A metal shroud surrounds the vertically affixed camera, which contains an opening large enough for the lens to fit through while allowing access to the rear of the camera for cable management and removal. The shroud provides a positive effect on the running temperature of the sensor within the hyperspectral camera, reducing potential noise.
What are some interesting/novel technologies you’ve deployed recently that you think could become more popular?
Tactile and hyperspectral systems for mammary tumor characterization, work I did in collaboration with Temple University Hospital and University of Pennsylvania Hospital doctors, was quite interesting. Typically, routine mammograms detect malignant breast tumors—a process that exposes the patient to potentially harmful radiation. A biopsy of suspected malignancies then confirms the presence of a tumor. Because those procedures require a large hospital setting with dedicated operators, many women have limited access to this important and often life-saving diagnostic.
An inexpensive, simple-to-use patient-centric tumor screening system allows many more patients to conveniently identify potential malignant tumors at early stages. Tactile imaging measures the elastic modulus of a tumor while hyperspectral imaging detects different biomarkers. Primary health care providers may use this device during clinical breast cancer examinations for accurately detecting small malignant tumors at early stages. This relatively simple device could be used in offices of primary care physicians where the accessibility is much greater due to proximity and convenience—which is especially beneficial to patients in rural and remote regions with limited access to large hospitals. This project has the potential to change the malignant tumor screening paradigm from a large hospital-centric to patient-centric model not only in the US but around the globe. Clinical studies have been performed on human patients and the device is currently being commercialized.
I also pioneered a hyperspectral imaging method for canine mammary tumor characterization, which was done in collaboration with University of Pennsylvania Veterinary Hospital. Among domestic species, canines have the highest occurrence of mammary cancer. This technology can provide veterinarians with a non-invasive imaging method to determine whether surgery is necessary, or monitoring is a reasonable alternative. This is particularly useful in older dogs with concurrent health issues.
Another recent project of mine is a hyperspectral imaging-based contamination detection system. Foreign body contamination is recognized as one of the most common reasons for recall of products like food, tobacco, etc. Complying with requirements for product safety and maintain consumer confidence, requires rapid, non-destructive techniques for foreign body detection and identification in agricultural products. To meet these goals, I created a real-time contaminant detection method using hyperspectral imaging technology. Glass, metal, plastic, foam etc., are the most frequently cited foreign bodies in processed foods. Metal detectors are commonly implemented in food processing chains to prevent metal fragments occurring in finished products; however, these instruments are not capable of detecting other contaminants.
The system incorporates a hyperspectral imaging system operating in the near-infrared region (900 to 1700 nm). The hyperspectral imaging system continuously scans the sample on the conveyor belt and captures a spectral image containing tobacco being processed and transported on the conveyor, which may contain impurities like foam, cardboard, plastic, etc. The system works under the principle that subtle differences in the hyperspectral images exist between different materials. Our team developed an algorithm that identifies these differences (called spectral fingerprints) and uses these to separate the impurities or undesirable materials from the sample flow in real time on the conveyor belt.
Since the detection of contaminants is completely automatic, it eliminates the human subjectivity and error. This technique could also apply to the classification and identification of the common contaminants in industrial processing systems and could be used in a wide variety of industrial applications like food processing, agricultural systems, and other manufacturing environments. Several companies have inquired about this system and are interested in implementing it.
Furthermore, I recently developed a method for the non-invasive detection of microscopic defects in organic coatings on metal substrates using fluorescent microscopic hyperspectral imaging. Detection of micro-cracking of the enamel applied to the underside of metal substrates represents one such example. The system incorporates a microspectrophotometer that operates in the UV-visible-NIR region (250 to 900 nm). The system works on the principle that the metal surface does not exhibit any fluorescence, while the coating material exhibits strong florescence when excited by UV and near UV energy source. We developed in-house algorithms that identify the differences in the spectral signatures and use these to identify microcracks on the metal substrates. This technology also has potential in crack detection of solar cells, and researchers around the world have inquired about the technology.
What is one prediction you have regarding machine vision in 2020?
In 2020, advanced robotic systems coupled with deep learning technologies will play an important role in the future development of machine vision systems. The availability of faster and cheaper hardware and sophisticated software tools will further aid this development. In 2020, as the pandemic hit almost every part of the world and social distancing became the new norm, machine vision systems have seen extensive use. Deep learning models help provide decision support for public health officials, thermal imaging cameras have been used in airports for temperature screening, and sanitizing robots have been used to clean hospitals. I am excited to see the field develop in the next decade and look forward to contributing to the growth.
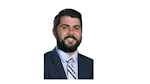
James Carroll
Former VSD Editor James Carroll joined the team 2013. Carroll covered machine vision and imaging from numerous angles, including application stories, industry news, market updates, and new products. In addition to writing and editing articles, Carroll managed the Innovators Awards program and webcasts.