Omnidirectional depth and stereo camera designed to improve upon traditional stereo cameras
A new technology based on a unique lens architecture is designed to replace using multiple stereo vision cameras or LiDAR to allow 360° of situational awareness.
For autonomous vehicles and robots, the fullest understanding by the machine of its environment is desirable or imperative. Inspiring consumer confidence in self-driving cars, for example, may prove difficult if the vehicle is not at least as competent as a human driver who uses side-view and rear-view mirrors to see. LiDAR systems and the full 360° views they generate were one of the earliest technologies employed by autonomous vehicle developers.
Coupling LiDAR with complementary technologies such as stereo cameras provides increased fidelity and redundancy for depth perception and object location. This requires additional compute power.
Stereo cameras, a more affordable option generally than LiDAR for 3D imaging, replicate the human eye by capturing left and right views of an object. Resolving the baseline disparity between the images provides the necessary data for producing depth maps or 3D point clouds. Also like the human eye, stereo cameras have a limited field-of-view (FoV), such that in some applications a full 360° of situational awareness may require multiple cameras.
The PAL camera developed by DreamVu, on the other hand, senses in full 360° at all times by using a proprietary stereo lens. Each petal on the stereo lens is a mirror (Figure 1). When light enters the body of the camera, it strikes and bounces off the petals. It must strike at least two petals to create a final, stereo image of that point. The two vectors generated by the same point strike the CMOS sensor at two different places, creating stereo left and stereo right views of that point.
Depending on the placement of the point relative to the position of the stereo lens, the light from that point may strike multiple petals and create multiple vectors. In this case, even though the light will strike the CMOS sensor at more than two locations, an algorithm in the DreamVu software resolves the multiple vectors from that point into two positions on the sensor, left and right. The more vectors the CMOS sensor receives from that point, the higher the resolution of the final stereo image of that point.
All of the points taken together create the stereo left and stereo right images of the panoramic scene captured by the camera. The resolution of the disparity between the two images creates a panoramic RGB image and a panoramic depth map (Figure 2).
The raw captured images are warped. To produce the stereo RGB images and corresponding depth map, the PAL camera’s software dewarps the image using proprietary computational imaging routines. According to DreamVu, this process is more compute-friendly than stitching together two narrow-field stereo images to create an image with a larger view.
For the PAL camera and smaller PAL Mini model, the base software runs on the host platform. The cameras use USB 3.0 interface to transmit data and draw power. The minimum platform specifications include an Intel i5- or i7-series processor and 2 GB of memory. DreamVu benchmarks using an i7-8700 processor and according to Mark Davidson, Chief Revenue Officer, the device never occupies more than 200 MB of RAM and uses approximately 300M CPU cycles at highest resolution.
A third model, the PAL Ethernet, features GigE interface and an integrated NVIDIA Jetson Nano platform. The dewarping process in this case takes place within the camera instead of on the host platform. The PAL Ethernet model also requires dedicated power from the host device.
Related: Recent advancements expand the scope of 3D imaging for machine vision
The PAL camera features IP67 protection and captures RGB and depth information at 9.5 MPixel resolution at 10 fps, 4.3 MPixel resolution at 20 fps, 0.6 MPixel resolution at 40 fps, and 0.15 MPixel resolution at 100 fps. At highest quality, the angular resolution is 0.068° horizontal and 0.060° vertical. Objects 2 × 2 cm in size up to 10 m away will show up in the depth map. The software may not recognize precisely how far away an object at this distance and may provide an estimate between 9 and 11 m. Accurate distance gauging begins at 5 m and at a distance of 1 m the camera can accurately measure tight spots and how to navigate around them. The PAL Mini has a maximum depth range of 3 m.
Stereo cameras depend on the presence of objects in their FoV to create disparity and calculate depth. When facing a completely blank, featureless white wall they likely cannot register the distance to the wall. A full 360° view affords the PAL camera greater opportunity to detect this texture information somewhere in its field of view, for instance the seam where a white wall and a white ceiling meet.
Each lens of a stereo camera has a separate FoV and the ability to calculate depth begins at the position where they intersect. This creates a near-field blind spot that necessitates specific working distances. The PAL camera, by virtue of not depending on independent lenses, lacks this near-field blind spot.
Even with its relative advantage regarding FoV limitations, some applications may require multiple PAL cameras. Autonomous mobile robots used in warehouses for logistical applications like picking or pallet transport may require two PAL cameras, one mounted on the front-left corner and one mounted on the back-right corner of the robot, each with a 270° FoV, to eliminate blind spots. DreamVu suggests that deploying two PAL cameras is more compute-friendly than having to process four stereo feeds simultaneously, however.
This configuration is designed to allow faster safe speeds, greater agility, and more efficient maneuvering for autonomous robots. A robot equipped with multiple stereo cameras may have to stop and turn several times before leaving one aisle of a warehouse, for example, and proceeding down a neighboring aisle. According to DreamVu, an autonomous robot equipped with a pair of PAL cameras could make a single, smooth turn.
The company also touts the potential benefits for simultaneous localization and mapping (SLAM) and obstacle detection and obstacle avoidance (ODOA) applications owing to the PAL camera’s lack of blind spots, omnidirectional point clouds, and ability to capture entire scenes in each frame. DreamVu also suggests the PAL camera may provide benefits for applications like automated cleaning or hospital disinfection while requiring less compute than other options.
DreamVu offers an OpenCV- and Robot Operating System (ROS)-compatible SDK that requires Ubuntu 16.04+ and a package of plug-and-play modules for human detection, ODOA, scene segmentation, 3D mapping, and object recognition routines called Vision Intelligence Software (VIS). This software is not required to run the PAL camera. Running VIS requires dedicated GPUs.
The PAL camera is currently deployed for several applications. DreamVu cannot disclose the names of its customers nor the specific applications in question.
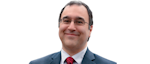
Dennis Scimeca
Dennis Scimeca is a veteran technology journalist with expertise in interactive entertainment and virtual reality. At Vision Systems Design, Dennis covered machine vision and image processing with an eye toward leading-edge technologies and practical applications for making a better world. Currently, he is the senior editor for technology at IndustryWeek, a partner publication to Vision Systems Design.