Autonomous search-and-rescue drone sees through forest canopies
Aerial searches have the potential to locate persons lost in the woods much faster than manual ground searches. Thick forest canopies make it difficult to spot human beings from the air, however. The greater the number of search flights, the higher the chance of locating a lost person. Human endurance limits the number of times any pilot can fly without needing rest. Search-and-rescue operations are also very time-sensitive, as the longer a person remains lost, the greater the risk of dehydration or injury by exposure.
Researchers from the Computer Science Department at Johannes Kepler University (Linz, Austria; www.jku.at/en) describe in their paper titled “An autonomous drone for search and rescue in forests using airborne optical sectioning,” a system that mounts a thermal camera onto an unmanned aerial vehicle (UAV), uses machine vision to analyze the footage, and executes movement autonomously to conduct the most efficient searches possible.
The system consists of a MikroKopter (Moormerland, Germany; www.mikrokopter.de/en) Okto XL 6S12 UAV; Teledyne FLIR (Wilsonville, OR, USA; www.flir.com) Vue Pro camera with 9 mm fixed focal length lens imaging on a spectral band of 7.5 to 13.5 µm; a Raspberry Pi 4B system-on-chip (SoC); Intel (Santa Clara, CA, USA; www.intel.com) Neural Computer Stick 2; and a Sixfab (San Jose, CA, USA; https://sixfab.com/) 3G/4G/LTE communications module, all mounted on a rotatable gimbal with the camera pointed downwards during flight.
The SoC controls the drone’s movements and triggers the thermal camera, downloads and preprocesses the recorded images from the camera’s memory, and computes the final image, all while the UAV continues its search pattern.
A process called Airborne Optional Sectioning (AOS), a set of computational imaging algorithms developed by the researchers, effectively removes the forest canopy from the raw thermal camera images. This allows the UAV to see the forest floor. Deep learning classification algorithms then process the thermal images to detect the heat signature of human beings.
While the UAV accepts programmed movement patterns, it can autonomously make pathing decisions to sweep areas after detecting a human presence on a first pass to verify the results. If the UAV detects a human, a message transmits via the communication module to alert rescuers.
The message contains the image the drone computes using AOS (the thermal image of the detected person), the GPS coordinates where the person was found, and the neural network’s confidence score that the location is correct. The UAV otherwise does not require a link to any communications network. This minimal data-relay technique allows the system to operate effectively even in areas with sparse network coverage.
The researchers tested the system in 17 field experiments, with flights conducted over varied types of forest and varied flight conditions (clear skies, rain, etc.). For missions where the UAV conducted straight flight paths, average precision measured at 86%, discovering 30 of 34 persons. When the UAV made autonomous flight decisions, it discovered 8 of 8 hidden persons and classification confidence increased by 15% compared with the searches with predefined flight paths.
About the Author
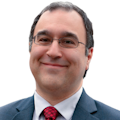
Dennis Scimeca
Dennis Scimeca is a veteran technology journalist with expertise in interactive entertainment and virtual reality. At Vision Systems Design, Dennis covered machine vision and image processing with an eye toward leading-edge technologies and practical applications for making a better world. Currently, he is the senior editor for technology at IndustryWeek, a partner publication to Vision Systems Design.