How 4D Imaging Radar is Propelling Autonomous Vehicles Forward
As the world hurtles toward an era of autonomous vehicles, the search for safer, more reliable sensors has reached unprecedented heights. At the forefront of R&D lies 4D imaging radar.
With its ability to provide real-time, accurate insights into the surrounding environment, 4D imaging radar is poised to become a key technology for the autonomous driving industry, providing data that complements the output of other sensors, particularly cameras.
In this article, we examine the history and science behind radar development, the varied applications of different radar systems, and the impact of 4D imaging radar on autonomous vehicle technology.
A Developmental History of Imaging Radar
Early radar systems were developed in the late nineteenth and early twentieth centuries. These first radar systems could measure the position and direction of moving objects and convey this information as numerical data to operators either acoustically or via an A-scope display (also known as a range scope).
In the 1950s, the invention of B-scope displays allowed operators to view a 2D top-down representation of the objects they were monitoring in the first practical application of imaging radar. This innovation would go on to be used in different types of displays that provided data output of range and azimuth for direction, as well as elevation in some cases.
2D radar—generally the cheapest kind of radar available and still used today—is useful for any kind of application that only requires range tracking. For example, it is still used as an early warning system for airspace control.
3D radar solutions became common by the 1960s and 70s, packaging range, azimuth, and elevation data output in a much more convenient and efficient display output. This advancement was made possible by either steering a radar beam through a scan pattern to build a three-dimensional image, or with “stacked beam radars” that compare the data of multiple radar beams from two or more elevation angles.
3D radar improves upon 2D systems in most respects. For example, whereas early WW2 air surveillance technicians had to operate two separate radars to properly locate enemy aircraft, 3D radar now makes this possible with a single system. These systems are more expensive, however, and are thus only used in applications where full three-dimensional positioning data is required.
In recent years, the concept of stacking radar beams has been expanded upon to build 4D imaging radars that also output velocity in addition to the data provided by standard 3D radars. These radars use Multiple Input Multiple Output (MiMo) antenna arrays that measure time-of-flight between many antennas. By using numerous antennas, the 4D imaging radar can gather enormous amounts of data to generate a precise three-dimensional ‘point cloud’ model of a target object or environment.
4D imaging radar enables simultaneous detection, mapping and tracking of multiple moving targets in high definition. It is one of the key technologies being developed to enable higher levels of autonomy in self-driving vehicles.
Related: Thermal Ranging Technique Delivers Detailed Images
How Radar Systems Operate at Different Frequencies
In addition to 2D, 3D and 4D output parameters, radar systems are categorized according to their frequency band. Radar systems calculate a target’s position by sending out a pulsed signal and measuring the elapsed duration by which the signal returns. Lower frequency signals have a longer wavelength—meaning the signals travel a longer distance before bouncing back to the receiving antenna. The inverse is also true, with higher frequencies having very short wavelengths that travel shorter distances.
This classification is important because it imparts different benefits and disadvantages to different kinds of radar systems:
- Lower frequency radar systems have a much longer range, but also require larger antennas.
- Higher frequency radar systems provide much faster and more precise data output, at the expense of shorter range.
Most industrial radar systems operate between 60 and 64 GHz. At this frequency, radar becomes more sensitive to small movements, enabling bespoke solutions to be developed for industrial environments that accurately monitor the movement of people.
Automotive imaging radar systems for driving assistance typically operate at 77 or 79 GHz. These frequencies are fast enough to provide the split-second reaction time required for autonomous vehicles and are usually accurate at distances up to 250 meters.
Very high frequencies become problematic because they can be blocked by water molecules. Some full-body scanners make use of this anomaly by employing 122 GHz radar systems—at this frequency, the signal can still penetrate clothing but is blocked by the moisture in human skin.
There are a few other kinds of radar systems that are useful for high-resolution imaging and precise tracking. For example, ultra-wideband radar (UWB) uses a broad frequency spectrum from 3.1 to 10.6 GHz and is commonly used for parking assistance systems. Millimeter-wave radar (mmWave) operates within an even broader frequency range from 24 GHz to 300 GHz and is also often used in Advanced Driver Assistance Systems (ADAS) systems for vehicles.
The above frequency bands can be combined with 2D, 3D and 4D radar systems, giving engineers a massive range of options.
Related: Depth Cameras Can Fill LiDAR's Autonomous Vehicle Blind Spots—Here's How
Practical Use Cases of Imaging Radar
Imaging radar has a wide range of applications that span across multiple sectors. Its use cases are diverse, and many different industries have found ways to employ the technology to solve complex problems.
Some common uses of imaging radar include:
- Monitoring crop growth, detecting diseases, assessing soil moisture levels, and estimating crop yields. It also helps during floods to monitor the on-ground situation and predict floods by tracking changes in water levels and soil moisture.
- Monitoring forest health, tracking deforestation, mapping wetlands, and measuring changes in glaciers to find out the impact of global warming.
- Assessing the situation after an earthquake, landslide, flood, or for search and rescue operations in remote locations.
4D Imaging Radar vs. LiDAR vs. Camera Sensors for Autonomous Driving
While dozens of industries can benefit from imaging radar systems, its application in the automotive industry—in conjunction with cameras—has the potential to help drive autonomous vehicles to higher levels of autonomy.
Video cameras were the first kind of ADAS sensor to kick off research into autonomous driving technology. The first ADAS system to use video cameras was the backup camera, which allows drivers to see directly behind the vehicle when parking or driving in reverse. Cameras are widely used today in ADAS, combined with video analysis AI systems trained to recognize traffic signs, road markings, pedestrians and obstructions. Multiple camera feeds can also be combined to create a three-dimensional representation of a vehicle’s exterior.
LiDAR is another kind of positional tracking sensor that uses directed laser beams at frequencies generally exceeding 600 THz. These systems can be equipped with over 100 lasers to create a 3D point cloud, like 4D imaging radar. LiDAR offers a very high degree of laser-like accuracy when compared to traditional radar. However, LiDAR has a serious drawback: It tends to be very unreliable in adverse weather conditions such as fog and rain, making it a liability for driving applications. LiDAR is also more expensive than radar.
The automotive industry is now focusing on 4D imaging radar technology, which has no weather limitations, to replace vehicles’ front and back sensors. Conventional but higher resolution radar would be used for the corners of vehicles. Furthermore, 4D imaging radar can provide additional or extended data beyond LiDAR’s primary design, including the accurate distance, azimuth, relative speed, and height of an object above the road.
Combining the use of 4D imaging radar and cameras increases vehicles’ level of autonomy; reliance on LiDAR would be reduced to instances where there might be a need for redundancy and verifiable safety.
A key feature of 4D imaging radar is how it sends out dense signals in all directions, enabling it to detect the position of occupants inside the vehicle and even differentiate between a toddler and an adult. This feature has multiple safety-related use cases, such as detecting intruders in the car or vicinity, providing advance seat belt warning, deploying airbags, and more. Its capability to detect the height of an object also allows it to warn truck drivers well in advance if their vehicle can’t pass through a particular underpass bridge.
4D radar can detect objects accurately and distinguish between different types of obstacles on the road. This new radar technology has already been applied in vehicles to improve safety with multiple features such as blind spot detection and lane change and parking assistance. Many tech companies are leveraging this technology to come up with next-generation imaging solutions.
Related: Centralized Radar Architecture Improves Perception Capabilities
The Future of Imaging Radar
Autonomous driving is currently at Level 3, which means while cars can drive on their own, they still need a human presence in the car.
The auto industry is working on improving imaging radar technology so that Level 4 and 5 driving automation can become a reality and vehicles can drive on their own without any need for a human presence.
For vehicles to reach this Level 5 autonomy, low-level sensor fusion algorithms must be developed that allow data from 4D imaging radar to be integrated with other sensors, such as cameras. Once this is possible, data from different sensors will work in tandem, allowing the vehicle to make real-time decisions based on millisecond-by-millisecond changes in road conditions.
Wider aperture radar systems are also required for vehicles to accurately classify every single object they “see” while driving. To make this possible, we need to find a way to integrate more transmit-and-receive channels on a car’s windshield than what is currently possible.
Advancements in imaging radar technology are now happening at a breakneck speed. With such a rapid rate of progress, it seems inevitable that in the not-so-distant future, we will soon see roads full of fully autonomous and driverless vehicles.
About the Author
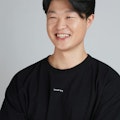
Jae-Eun Lee
Jae-Eun Lee is founder and CEO of bitsensing, a 4D imaging radar design company based in Seoul, South Korea. Before founding bitsensing, he was a senior research engineer at Mondo Corporation, a Tier 1 OEM automotive supplier. He has a PhD in electrical engineering from Seoul National University.