Cornell University study suggests stereo camera systems could replace LiDAR
Researchers at Cornell University have presented evidence that inexpensive stereo camera setups could provide nearly the same accuracy as the expensive LiDAR systems that are currently used in the most common approaches to developing autonomous driving technology.
In a new research study titled "Pseudo-LiDAR from Visual Depth Estimation: Bridging the Gap in 3D Object Detection for Autonomous Driving," the authors describe a new technique by which to interpret data gathered from image-based vision systems. This new form of data interpretation, when fed into algorithms that normally are used to process information gathered from LiDAR systems, greatly increases the accuracy of image-based object detection.
The need for deploying multiple sensor systems in autonomous vehicles is understood, as demonstrated by recent initiatives like the KAMAZ autonomous truck project and TuSimple's pairing of LiDAR, radar, and camera systems in its autonomous truck system. The need to address LiDAR's difficulty with fog and dust is also the subject of recent research at MIT. The Cornell study suggests that, at the very least, stereo camera systems could provide inexpensive backup systems for LiDAR-based detection methods.
The quality of the point clouds generated by LiDAR and stereo camera depth estimators are not dissimilar, argue the researchers. However, algorithms that use image-only data achieve only 10% 3D average precision (AP), whereas LiDAR systems achieve 66% AP, as measured by the KITTI Vision Benchmark Suite developed by the Karlsruhe Institute of Technology and Toyota Technological Institute at Chicago.
The researchers suggest representation of this image-based 3D information, and not the quality of the point clouds, is responsible for LiDAR's comparatively-superior performance. LiDAR signals are interpreted into a top-down, "bird's-eye view" perspective, whereas image-based data is interpreted in a pixel-based, forward-facing approach that distorts object size at distance and thus makes 3D representation more difficult the further away the data was gathered from the cameras.
The solution hit upon by the Cornell researchers was to convert the image-based data into a 3D point cloud like that produced by LiDAR, and to convert the data into a bird's-eye view format prior to feeding the data into a 3D object detection algorithm normally used to interpret LiDAR data. 0.4 MPixel cameras were used for the experiment. The result still did not equal the 66% AP achieved by LiDAR. The AP of the image-based data was improved to 37.9%, however. The researchers suggest that higher-resolution cameras may improve the results even further.
Wholesale replacement of LiDAR by stereo camera systems is not yet achievable but, according to the research published by Cornell, is hypothetically possible. The researchers further suggest that if LiDAR and image-based systems were both present on the same vehicle that the LiDAR data could be used on a consistent, ongoing basis to train a neural network designed to interpret image-only based 3D data, thus improving the accuracy of image-based systems as backup for primary LiDAR systems.
(All images courtesy of Lindsay France/Cornell University)
Related stories:
Novel thermal imagers fill gap in autonomous vehicle sensor suite
Researchers at MIT develop system to assist autonomous vehicles in bad driving conditions
Russian project to develop fleet of self-driving delivery trucks is underway
Share your vision-related news by contacting Dennis Scimeca, Associate Editor, Vision Systems Design
SUBSCRIBE TO OUR NEWSLETTERS
About the Author
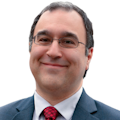
Dennis Scimeca
Dennis Scimeca is a veteran technology journalist with expertise in interactive entertainment and virtual reality. At Vision Systems Design, Dennis covered machine vision and image processing with an eye toward leading-edge technologies and practical applications for making a better world. Currently, he is the senior editor for technology at IndustryWeek, a partner publication to Vision Systems Design.