Could vision-guided robots be key to keeping the restaurant industry afloat?
Restaurants have suffered perhaps more than any other industry in the United States from the COVID-19 pandemic. Local and national guidelines have seen restaurants running limited operations or closing indefinitely in many cases. Enter vision-guided robots and machine vision, which offer great potential for certain tasks and types of restaurants.
Paul Goethals, Business Development Manager, Agro Food Robotics, Wageningen University and Research Food & Biobased Research (Wageningen, Netherlands; www.wur.nl/food-and-biobased-research) works with robots in the food production chain from primary production, storage, logistics, and food processing. Goethals, and others in this article, suggest that vision-guided robots and machine vision could help restaurants.
“With the coronavirus situation and restaurants being closed, the thinking is less human-to-human interaction, which will give way to robots,” he says. “Opportunity exists for serving, pick-up, and cleaning tasks in restaurants, and technologies like simultaneous localization and mapping (SLAM), 3D stereo vision, and LiDAR could help provide real-time environment mapping and navigation.”
Fast food
Not all restaurant types are a natural fit for deploying vision-guided robots, but fast food establishments may represent the optimal environment, suggests David L. Dechow, Principal Systems Architect, Integro Technologies (Salisbury, NC, USA; www.integro-tech.com).
“Fast food likely represents the biggest opportunity due to the repetition in the food they prepare and serve, and I think it could be quite successful in these environments,” he says. “Robots could cook hamburgers and could also be used for food preparation, for example.”
Machine vision technologies most likely to add value in fast food environments may include 3D Time of Flight, for tasks like locating and placing a pickle on a sandwich and placing items on a plate. Thermal imaging could also help keep an eye on when food is done, suggests Tom Brennan, President, Artemis Vision (Denver, CO, USA; www.artemisvision.com).
Related: 3D-vision-guided robots assist in COVID-19 measures in China
“These technologies could enable robotic tasks in fast food environments that may include automated slicing and cutting on pre-prepped food materials, applying toppings or spices,” he says. “Other tasks, such as picking up food items, removing items from packages, or trimming food products will still be difficult though.”
Of course, machine vision technology types that prove most useful depend on individual tasks. Robots greeting guests and guiding them to a table may only require simple machine vision technologies for navigation and obstacle avoidance. More complicated tasks like working with food necessitates the use of 3D vision, such as some of the commercialized vision sensors today, says Dechow.
“From both the robotics and machine vision side, deploying vision-guided robots in restaurants feels like a task for 3D vision products including or based on the Intel RealSense or Sony DepthSense,” he says. “Pairing such a low-cost sensor with a robot arm or mechanism and constraining the food production area could provide a sufficient means for some automated tasks.”
Peter Gwilliam, Chef de cuisine, Pastoral (Boston, MA, USA; www.pastoralfortpoint.com), who has worked under notable chefs Mario Batali, Todd English, and Paul Kahan agrees that fast, casual restaurants likely suit robots best. When visiting such places, he explains, people aren’t necessarily looking for an experience, but instead, seek reasonably priced food that tastes good and is ready in under 10 minutes. In this type of setting, robots could seat guests, while taking and delivering orders, he says.
Beyond helping to prop up or safeguard restaurants in the future, vision-guided robots may also assist restaurants faced with labor issues. In other words, not just cost reduction but substituting for unavailable labor, explains Perry West, Founder and President, Automated Vision Systems, Inc. (San Jose, CA, USA; www.autovis.com).
“Many locations exist where a McDonalds can operate profitably, but a lack of a labor pool exists,” he says. “For example, areas where the prevailing wage is much higher than what a McDonalds may offer, they will have trouble attracting enough staff for the franchise.”
He adds, “In general, for tasks like cooking and flipping hamburgers, machine vision might be a real asset.”
To that end, Miso Robotics (Pasadena, CA, USA; www.misorobotics.com) developed Flippy, an autonomous robotic kitchen assistant that learns from its surroundings and acquires new skills via deep learning techniques. Flippy, a vision-guided collaborative robot system, can cook food consistently every time and offers features such as automatic tool switching and cleaning, OSHA safety compliance, washdown compatibility, cloud-based monitoring and learning, and currently runs with 99.7% uptime.
The robot’s sensor suite includes OSHA-compliant LiDAR (light detection and ranging) sensors that allow staff to collaborate alongside it safely, 3D scanners and thermal cameras for vision, and a cloud-connected Miso AI for data sharing and cloud learning. Miso AI enables the robot to perform all its tasks, from detecting when raw burgers go on the grill, to removing the burger and wiping the surface of the grill with a scraper. In addition to the grill, Flippy can work at the fryer, capable of picking up baskets and placing them in a fryer and monitoring the process until it’s time to hang the baskets to drain excess oil upon completion (Figure 2).
“Flippy uses deep learning to locate and identify objects on the grill, as well as equipment and other objects in the kitchen,” says Dr. Ryan Sinnet, CTO and Co-Founder of Miso Robotics. “We have also developed advanced pose estimation technology using neural networks which allows Flippy to precisely identify the location of objects in the kitchen and pick them up with high reliability. The theoretical breakthroughs we’ve made were necessary to take low-reliability cutting-edge machine learning research and use it in a commercial environment.
Flippy also leverages machine learning to perform task scheduling. By doing so, the objective function can be modified to suit the needs of a restaurant. For example, if a restaurant wants to ensure customers get their food within three minutes, or if a restaurant wants to make sure all food in an order comes out at the same time so half the order isn’t cold, Flippy enables such activities, according to Sinnet.
Another important facet of the robot is data efficiency, as Flippy features a toolbox of data augmentation techniques that allows it to learn more from each piece of data. Flippy therefore continuously learns to recognize new foods with high reliability using only a couple hundred images instead of several thousand, says Sinnet.
“This is a key technology underlying the scalability of Flippy, which enables the kitchen assistant to quickly adapt to different restaurant menus,” he says. “We started with burgers and are now up to frying more than 14 different foods.”
Restaurants currently deploying Flippy include the fast-casual restaurant chain CaliBurger, with locations in Pasadena, CA, USA, and Fort Myers, FL, USA; as well as restaurants in Dodger Stadium and the Arizona Diamondbacks’ Chase Field. Additionally, more big brands could be on the way, explains Sinnet.
“Miso Robotics is in talks with several big brands looking at how they can address the challenges they are facing resulting from the pandemic,” he says. “Demand for automation is surging and we expect to announce new customers this year, with some very recognizable quick service restaurants.”
He adds, “Once restaurants begin to reopen widely, balancing safety, volume and scaling will be vital - they simply can’t survive without putting measures in place that allow them to stay efficient and reinforce their commitment to keeping pathogens out of the food service and delivery equation,” he says. “Flippy is NSF certified and Miso Robotics is always looking at how industry is demanding innovation to control the spread. At Miso Robotics, we are looking at how we accelerate and enhance a higher level of health and safety to help businesses reopen.”
Related: Vision-guided robot monitors and inspects hazardous environments
For example, Miso Robotics is looking into incorporating social distancing readings for the kitchen in which Flippy operates, while also exploring strategic partnerships and working with third parties to map out a successful plan for restaurants going forward. Automation remains a huge part of that, as it offers faster production for higher returns during business hours and consistent quality to keep takeout and delivery customers coming back, says Sinnet.
Fine dining
Fine dining restaurants may be much less suitable for vision-guided robots, outside of maybe cleaning-type tasks, suggests Gwilliam.
“One of my favorite things to tell young cooks is ‘you are not a robot,’” he says. “So many minute details go into each dish and each interaction with the guest requires people thinking for themselves. The same thing applies in front of house, as managers will often tell servers ‘don’t be an order taker, anyone can do that.’”
Gwilliam continues, “A server’s job involves connecting with the guest and ensuring they have a memorable experience. We tell our staff all the time that we are in the hospitality business and there is a difference between service and hospitality. Service is making sure a steak knife is on the table before the steak arrives and hospitality is how you make people feel.”
Agreeing with the sentiment, Dechow notes that it may come down to the social aspects of fine dining.
“I don’t see the potential for robotics in high-end restaurants, personally,” he says. “People go to such restaurants to be served great food and for the experience. If I didn’t want this, I could just do my best at making it myself at home. Socially, I just don’t see this happening.”
Real-world application and technical challenges
Someone as familiar as anyone on the topic is Josh Goldberg, Vice President of Research and Development, Zume (Mountain View, CA, USA; www.zume.com). While Zume’s current technology focuses on sustainable food packaging, the company previously sought to revolutionize pizza and other food delivery. Specifically, Zume wanted to provide artisanal, Neapolitan style pizza fresh at a customer’s door with delivery trucks fitted with pizza ovens.
Prior to getting the pizza ready for delivery, however, the company used vision-guided robots for robotic pizza assembly. Robots performed specific tasks that enabled human workers to do what they are best at – putting together a great tasting product, according to Goldberg. These duties included spreading sauce, stretching dough, working in and around the oven, and putting items onto racks.
“It’s really hard for restaurants to change, and resistance stems from the fact that margins are thin and its generally hard business being a restauranteur,” he says. “Nothing illustrates this better than the current situation. Occupancy costs are really high, and people can’t go occupy the restaurants at all.”
Material handling, according to Goldberg, represents the biggest technical limiting factor in using vision-guided robots in restaurants. A current, well-known pizza company uses a fully automated system with individual pieces of machinery for handling pepperoni, vegetables, cheese, and other ingredients, but the design did not suit Zume, he says.
“While a fairly impressive system, Zume desired a solution that did not use 100 ft. of preparation equipment to make 14 different types of pizza,” says Goldberg. “So, we left this part [toppings] to humans, since it is a hard material handling problem.”
He continues, “Identifying all the common things that must be done to all pizzas [sauce, dough, oven work] helped us decide which processes to automate.”
If a restaurant has a consistent product coming out of the kitchen every single time, automation may be a very valuable technology. Many or most restaurants have extensive menus that allow for customization. Even when consistent, this does not always mean exactly the same every time.
“In the case of pizza, a person may order no olives or extra pepperoni, and the gold standard image used for quality check will not hold up with customization. The combinatorial problem of what every pizza looks like, is massive, and building a training data set for every possible combination is impossible when a restaurant has a reasonable number of toppings or different varieties or combinations of meals.”
Goldberg also agrees with the sentiment that fine dining does not likely represent an ideal environment for automation, but fast casual restaurants do. The more consistent the product sold, the easier automation will be, he explains.
“Counting chicken nuggets in a box and ensuring they are all the same color brown is a relatively easy vision problem to solve,” he says.
Quality inspection in some fast-casual restaurants may prove difficult, but machine vision could still add value for tasks such as inventory monitoring, making sure bins are full, or even browning ground beef continually and moving it into a bin when ready. Such tasks do not require significant precision, but require consistency, and could be used suitably in such environments, he suggests.
“Ultimately, when dealing with handmade food, people can easily recognize what they are working with, but this is something that machines are still getting better at,” says Goldberg. “This is my number one lesson learned.”
Despite the current lack of commercialized vision-guided robots in restaurants, Goldberg still believes that there will absolutely be widespread adoption at some point.
“Machine learning classification continues to improve, and the research on how to adapt systems to unknown scenarios does as well. It is not impossible for widespread adoption of robots in restaurants, it is just not currently practical,” he says. “To get us to that point, the deep learning systems and classification systems need to improve even further to the point where systems can handle different adaptations without requiring thousands of samples for training. Restaurants can’t spend money on thousands of samples and do not want to hire programmers, either.”
In the past, this may have required an expensive, real-time camera system for inspection. Now, Goldberg provides as an example, Raspberry Pi offers a high-resolution camera that allows people to go all-in for $200.
“I can see systems like this being affordable and realistic for restaurants once the companion software is there.”
Government guidelines
Another factor to consider in deploying vision-guided robots or machine vision technology in restaurants is approval from the U.S. Food and Drug Administration (FDA), which sets the Food Code that city and state Departments of Health use when inspecting local businesses. This code aims to keep food at safe temperatures and conditions as to prevent foodborne illness and the spread of disease. For example, collaborative robots from Universal Robots (UR; Odense, Denmark; www.universal-robots.com), are not FDA approved in the United States. But in Europe, UR robots perform related tasks like specialty food packaging in Northern Europe, picking and packing eggs in Italy, and quality control on cured meats in Spain (Figure 3).
Related: Vision system inspects, counts bakery products
“An interesting spin on the current situation with restaurants is FDA approvals,” says Steve Varga, Principal Scientist, Sensing Measurement and Automated Quality R&D, Procter & Gamble USA (Cincinnati, OH, USA; www.pg.com). “A lot of machine vision projects are being requested for food and medical applications that were never considered before, and food service is just one example.”
He continues, “It would be great to see some practical guidelines for the machine vision space on navigating FDA, ISO, and IEC standards. Helping machine vision professionals know where to turn for help could improve the safety for everyone and make a world safer to return things closer to normal.”
Financial practicality
Widespread restaurant adoption of vision-guided robots depends on the potential for helping the bottom line. Ultimately the technology will be embraced by the industry if it makes financial sense. After all, it is the restaurant business.
Two considerations underline the key question of whether vision-guided robots could be key to keeping the restaurant industry afloat, Dechow says.
“Is there a reasonable return on investment for deploying robots in restaurants, and can the technology be simplified or commercialized so that it becomes easy enough for a restaurant manager to keep it working?” he says. “These represent the big questions, at least with today’s technology, as the robot needs to essentially be as easy to use as an alarm clock.”
He continues, “Can the robot and machine vision be that reliable, technically? Robots aren’t really that cheap yet, either. Combining the cost with the technological hurdle, the immediate challenge—both today and in the next 10 years—is the real hurdle to acceptance and putting robots into use.”
Brennan agrees, noting that he does not see implementations on the timeline of the coronavirus likely, but automation could help make businesses pandemic-proof in the future, in a lot of ways.
“The only real way to do this is to work with a company over time through a few stages of prototypes,” he says. “There will definitely be hidden problems beneath the surface that must be worked out.”
In the end, the commercialization of robots for consumer applications such as restaurants may not be ready just yet, but the concept is certainly not far-fetched. It will take a lot of money and dedication, but with time and effort, the eventual, successful implementation seems likely, according to Dechow. An accurate answer to the question of could vision-guided robots be key to keeping the restaurant industry afloat, is essentially yes, but the better question may be, will they? Eventually, perhaps.
About the Author
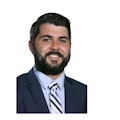
James Carroll
Former VSD Editor James Carroll joined the team 2013. Carroll covered machine vision and imaging from numerous angles, including application stories, industry news, market updates, and new products. In addition to writing and editing articles, Carroll managed the Innovators Awards program and webcasts.