Deep learning improves defect detection in complex applications
Next month, many of us will attend VISION 2018 in Stuttgart, Germany. During the three-day event, the global vision and imaging community will gather to discuss the latest machine vision applications, as well as emerging trends and technologies. According to Florian Niethammer, VISION Project Manager at Messe Stuttgart, one of the technologies expected to be featured prominently at the show is deep learning. Oddly enough, four of the eleven feature articles in this issue cover deep learning to someextent.
First, on page 15, Nuria Garrido López examines how deep learning helps Continental detect defects that were formerly undetectable. The company has been applying deep learning technology in inspection applications where the criteria that distinguish good part from bad vary strongly and defy numeric quantification.
Next, on page 32 we have an article by Jon Vickers that not only describes the difference between machine learning and deep learning, but also discusses how supervised learning methods can offer inherent advantages over convolutional neural networks.
The article on page 36 is all about the ability of photometric vision systems to tackle some of the most challenging assembly verification applications. In the article, Hubert Kostal and Anne Corning compare traditional machine vision, human inspection, photometric, and machine learning techniques being applied by many manufacturers in applications that typically fall into one or more of the followingcategories:
- Small, subtle, and low-contrastdefects
- Complex assemblies, with many components anddetails
- Random and unpredictabledefects
- Defects that need to be quantified, trended/analyzed, and addressed operationally via system or processimprovements
Finally, on page 41, Patrick Chabot explains how machine vision data management software simplifies root-cause analysis. In addition to explaining how cross-process analysis of images, image data and other process data improves quality, process control, and enables continuous process improvements, Chabot discusses how applying artificial intelligence requires data from which to learn, and that by making the data accessible and integrated, data management optimizes the potential of machinelearning.
As developers increasingly apply deep learning and artificial neural networks to improve defect detection and object classification, it is my hope that our coverage in this issue of the technology and its applications will help you better understand deep learning and stimulate many interesting discussions. As always, I hope you enjoy this issue.
About the Author
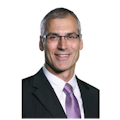
John Lewis
John Lewis is a former editor in chief at Vision Systems Design. He has technical, industry, and journalistic qualifications, with more than 13 years of progressive content development experience working at Cognex Corporation. Prior to Cognex, where his articles on machine vision were published in dozens of trade journals, Lewis was a technical editor for Design News, the world's leading engineering magazine, covering automation, machine vision, and other engineering topics since 1996. He currently is an account executive at Tech B2B Marketing (Jacksonville, FL, USA).
B.Sc., University of Massachusetts, Lowell