Open-source deep learning model extracts street sign locations from Google Street View
A new research study in service to local government from a trio of scientists at RMIT University in Australia uses techniques resembling those employed by Mapillary and Waymo but using open-source deep learning software.
The purpose of the research study, titled "Detecting and mapping traffic signs from Google Street View images using deep learning and GIS," was to assist local governments in improving their asset management of street sign inventories. The researchers investigated whether Google Street View (GSV) imagery could replace costly and time-consuming manual surveys, and whether a convolutional neural network (CNN) could be trained to review the GSV images and not only detect the presence of street signs, but also use photogrammetric analysis to determine precisely where the street signs stood.
The Melton and Melton South localities were chosen for the research area as the GSV data from this area was gathered in 2014, meaning that modern vehicles in Google’s fleet equipped with R7 capturing equipment, with a pixel size of 8.8 µm and focal length of 5.1 mm, would have captured the GSV imagery, which in turn meant the images would be of appropriate resolution for the study.
The researchers decided to focus their tests on detecting street signs located at intersections, these being the most important locations at which street signs must be posted and maintained in order to provide road safety.
To build their training dataset, the researchers used the relatively-complete street sign location dataset from the geographic information system (GIS) of the City of Greater Geelong (COGG), located southwest of Melbourne. GSV images were requested for the locations at which street signs were posted. RectLabel software was used to draw bounding boxes around Stop and Give Way signs that appeared in the GSV images, which created the training dataset.
Using the open-source machine learning platform TensorFlow, the researchers loaded the training dataset into an SSD MobileNet CNN model, and trained the model by tasking it to detect Stop and Give Way signs within the images. Training was performed on a cloud-based virtual machine, with a Quadro M4000 8 GB GPU, 30 GB of RAM, and 8 CPUs. The training process took four hours.
GSV images within 12 m of every intersection within the Melton and Melton South localities was then requested. This resulted in a dataset of 2382 images. The model achieved 95.63% accuracy in detecting the Stop and Give Way signs within the GSV images.
To determine whether photogrammetric analysis could be used to calculate the precise location of street signs identified by the CNN, the researchers again used the GSV image set based on the COGG’s street sign locations, because the ground-truth locations of those signs was contained within the city’s GIS.
Determining the accuracy of the CNN’s results was challenging, because some of the information within the city’s GIS proven to be inaccurate. However, the CNN was able to chart the real-world location of the street signs within what the researchers considered an acceptable tolerance for asset management purposes.
While the research had nothing to do with autonomous driving systems, the results demonstrated the potential to achieve street-sign mapping algorithms, the likes of which are being developed commercially and which can improve the safety of autonomous driving systems, without relying on proprietary software.
Images via Campbell, A., Both, A., & Sun, Q. C. (2019). "Detecting and mapping traffic signs from Google Street View images using deep learning and GIS." Computers, Environment and Urban Systems," 77, 101350.
Related stories:
Cornell University study suggests stereo camera systems could replace LiDAR
Researchers at MIT develop system to assist autonomous vehicles in bad driving conditions
LiDAR-based vehicle detection and classification system operates in free-flowing traffic
Share your vision-related news by contacting Dennis Scimeca, Associate Editor, Vision Systems Design
SUBSCRIBE TO OUR NEWSLETTERS
About the Author
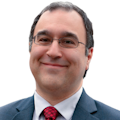
Dennis Scimeca
Dennis Scimeca is a veteran technology journalist with expertise in interactive entertainment and virtual reality. At Vision Systems Design, Dennis covered machine vision and image processing with an eye toward leading-edge technologies and practical applications for making a better world. Currently, he is the senior editor for technology at IndustryWeek, a partner publication to Vision Systems Design.