Satellite images analyzed by neural networks to determine rooftop solar power potential
A new system named DeepRoof analyzes satellite imagery with convolutional neural networks (CNNs) to calculate solar energy potential and can eliminate the need for solar potential evaluations.
Solar potential surveys are a manual process that determine whether sunlight strikes a rooftop with enough intensity to make worthwhile the installation of solar panels to generate electricity. Several attempts have been made remove the need for these on-site evaluations. The Mapdwell Solar System developed at the Massachusetts Institute of Technology generates 3D models of rooftops using high-resolution LIDAR and runs solar potential estimation algorithms against those models. Google’s Project Sunroof uses similar technology.
In a new research paper titled “DeepRoof: A Data-driven Approach For Solar Potential Estimation Using Rooftop Imagery,” scientists from the University of Massachusetts, Amherst and Microsoft Research in Bangalore, India argue that satellite information, which is less expensive to collect and covers a wider geographical range than LIDAR data, can also be analyzed by CNNs and employed for automated solar potential surveys.
DeepRoof can study all the buildings within a list of addresses or in an area defined by GPS coordinates, or focus on individual buildings. First, a neural network model for image segmentation called a feature pyramid network is used to analyze a satellite image and identify roof segments and their orientations and the locations of trees.Next, a topology estimation routine uses an outline of a target building obtained via the OpenStreetMap API and determines the roof geometry and orientation of the target rooftop from real-estate and Federal Emergency Management Agency (FEMA) data.
Solar irradiation data is calculated for each pixel in the image that has been identified by the CNN as a rooftop. The calculation accounts for roof orientation, the relative angle of the sun at different times of day, and shade from nearby objects. Finally, DeepRoof determines how many solar panels of a specified size could be placed on the rooftop in question.
Ground truth data was used to measure DeepRoof’s accuracy in predicting individual pixels as rooftop, which was around 91%, and accuracy in predicting flat or pitched roofs, which were >98% and >93% respectively. Two independent experts with experience conducting solar potential surveys scored an average of 8.8 out of 10 for DeepRoof’s accuracy in predicting solar potential estimates.
The researchers note that because Project Sunroof does not compare solar potential on a per-pixel basis, its solar potential estimates could not be meaningfully compared to the DeepRoof results. However, the available solar panel installation area results were within 11% of Project Sunroof projections.
Related stories:
Open-source deep learning model extracts street sign locations from Google Street View
Researchers develop set of natural images to break image classification systems
Cornell University study suggests stereo camera systems could replace LiDAR
Share your vision-related news by contacting Dennis Scimeca, Associate Editor, Vision Systems Design
SUBSCRIBE TO OUR NEWSLETTERS
About the Author
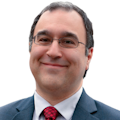
Dennis Scimeca
Dennis Scimeca is a veteran technology journalist with expertise in interactive entertainment and virtual reality. At Vision Systems Design, Dennis covered machine vision and image processing with an eye toward leading-edge technologies and practical applications for making a better world. Currently, he is the senior editor for technology at IndustryWeek, a partner publication to Vision Systems Design.