ShadowCam gives autonomous cars the ability to see around corners
Accident avoidance is one of the top concerns facing developers of autonomous vehicles. If full autonomy—or class 5 autonomy, literally having no human being at the wheel— is ever to become a reality, autonomous vehicles must be as entirely safe as possible. This means taking as many steps as possible against scenarios that could potentially lead to collision. Being able to see around corners would give an autonomous vehicle a large advantage toward achieving this end.
ShadowCam, a new technology developed by researchers at the Massachusetts Institute of Technology (MIT; Cambridge, MA, USA; www.mit.edu), working in collaboration with researchers from the Toyota Research Institute (Cambridge, MA, USA: www.tri.global) and with support from Amazon Web Services (Seattle, WA, USA; aws.amazon.com) demonstrates the potential to give advanced driver assistance systems (ADAS) the ability to read changes in illumination on the ground caused by dynamic obstacles—moving vehicles—that are not in direct line of sight.
The technology uses a region of interest technique, focusing the camera on the ground ahead of the vehicle, in the area where the appearance of a shadow cast by an approaching object is to be expected. The ShadowCam classifier pipeline uses a pre-processing routine to enhance images with weak signal, i.e. shadows. The algorithm then analyzes the images, comparing a pixel-based metric calculated from sequences of images against a safety threshold. If this metric is less than the safety threshold, i.e. if there are shadows, the vehicle is commanded by the algorithm to stop.
Two experiments were conducted, the first of which used an autonomous wheelchair moving down a hallway equipped with one of two different cameras, in different experiments. The first camera was a Canon (Melville, NY, USA; www.usa.canon.com) EOS 70D single-lens reflex (SLR) with EFS 17-59 mm lens, which was used in experiments where the position of the wheelchair and the shape of the hallway were denoted with AprilTag fiducial markers.
The second camera was a uEye UI-3241LE-M-GL monochrome, global shutter CMOS camera from IDS Imaging Development Systems (Obersulm, Germany; www.ids-imaging.com), which was employed when a direct sparse odometry method was used to determine the wheelchair’s environment and relative position.
Both cameras were tested against beyond-line-of-sight objects that were moving (dynamic) or stationary (static). The mean classification accuracy—successful classification of an object (dynamic or standing) beyond line of sight—was around 70%.
Experiments were also conducted using an autonomous Toyota (Toyota City, Aichi Prefecture, Japan; global.toyota/en) Prius driving in a garage. The illumination in the garage provided close to nighttime driving conditions in terms of the amount of illumination. The lights of the autonomous vehicle were kept off, in order not to flood the ROI in front of the vehicle with light. The Toyota Prius was equipped with the uEye UI-3241LE-M-GL camera, and the ROI and ground plane were also annotated for the ShadowCam algorithm.
The algorithm was able to detect the approach of a moving vehicle around the corner faster than a SICK (Düsseldorf, Germany; www.sick.com) LMS151 LiDAR sensor that was also deployed on the test vehicle.
About the Author
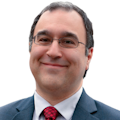
Dennis Scimeca
Dennis Scimeca is a veteran technology journalist with expertise in interactive entertainment and virtual reality. At Vision Systems Design, Dennis covered machine vision and image processing with an eye toward leading-edge technologies and practical applications for making a better world. Currently, he is the senior editor for technology at IndustryWeek, a partner publication to Vision Systems Design.