In 2018, the European Union adopted new guidelines for addressing the problem of textile waste, with the goal of completely recycling all textiles by 2025. Hyperspectral imaging deployed for quick and accurate classification of textiles for rapid sorting could make this goal achievable, according to research sponsored by SPECIM (Oulu, Finland; www.specim.com).
Manual textile sorting presents a slow, difficult, and expensive task. Workers also generally lack expertise on how to visually identify different types of fibers and substances with blended fabrics, which may produce inaccurate sorting results. Hyperspectral analysis and image classification may open the door to automated robotics sorting applications, however, by determining material types through chemical structure analysis.
The large number of different materials in mixed streams of textile products presents the primary challenge. Potential materials include animal fibers like angora, camel hair, silk, and wool; plant fibers such as cotton, flax, and hemp; and synthetic fibers like nylon, acrylic, and polyester. Blended fabrics may contain two or three different kinds of natural and synthetic materials.
Esko Herrala, co-founder and senior application specialist at SPECIM, collaborated on reports for the Finnish parliament that conclude hyperspectral imaging can identify all these types of materials and the proportions of different fiber types within blended fabrics.
Related: Sensor and camera requirements for hyperspectral imaging
To explore this potential use of its technology for textile sorting, SPECIM partnered with Prizztech (Pori, Finland; www.prizz.fi), a non-profit organization that coordinates with the Robocoast Digital Innovation HUB consortium (www.robocoast.eu), to conduct a study titled Textile identification and sorting using hyperspectral imaging (bit.ly/VSD_HYTXT).
Three SPECIM hyperspectral cameras were used in the experiment. An FX10-model camera captured wavelengths between 400 and 1000 nm, a Spectral Camera SWIR model imaged wavelengths between 1000 and 2500 nm, and an FX-17 camera captured wavelengths between 900 and 1700 nm. Two halogen light arrays, mounted on a SPECIM Labscanner 20 x 40 frame, were placed 70 cm above and at 45° angles to a 60 cm-wide simulated conveyor belt (Figure 2).
Image processing took place on a PC with Intel (Santa Clara, CA, USA; www.intel.com) i7 processor, 16 GB of memory, and SSDs for data storage. Images were acquired using SPECIM’s LUMO Scanner software. Geospatial ENVI from L3Harris (Melbourne, Florida, USA; www.l3harrisgeospatial.com), Perception Studio from Perception Park (Graz, Austria; www.perception-park.com), and SPECIM software provided quick analysis work.
A wide range of textile samples were gathered for the experiment, including clothing, upholstery, and knitting yarn. Some samples were new, and some were used and relatively old. Natural and artificial leather samples were also included. In addition, different colors of the same material, for instance cotton wool, were included in the sample selection, to test the effect on infrared imaging’s ability to identify different colors of the same base material.
Analysis at a wavelength range between 1300 and 1700 nm showed clear differentiation between polyester and cotton. Fabrics such as spandex or lycra that contain elastane, cellulose fibers, mixtures that had 5% or lower natural or synthetic material content, and very thin textiles were more difficult but not impossible to classify, (Figure 3).
The research determined that RGB cameras alone provided adequate images for color classification and separation. However, if the mix of materials to classify included the color black, analysis requires wavelengths above 1300 nm. Any material that included carbon as a dye, including black polyester, and black buttons and plastic zippers, made classifications using near infrared (NIR) wavelengths impossible. Even at wavelengths above 1300 nm, reliable classification of 100% polyester materials that were dark grey or black in color proved very difficult.
Surface structure did not generally affect the accuracy of material classification, with the exception of shiny materials with specular reflection that does not contain spectral information. Some areas of these materials provided enough information to make material classification possible but difficult. Materials with multiple layers were challenging to classify accurately unless all layers were clearly visible, i.e. as long as one layer did not lay entirely on top of and mask another layer.
Related: X-ray fluorescence scanner assists painting restoration
Water absorbs wavelengths in the NIR range. Wet textiles, therefore, can be difficult to classify with hyperspectral lighting. Cotton can absorb over 20 times its weight in water, making wet cotton very problematic for hyperspectral sorting applications. Synthetic materials such as polyester and nylon, on the other hand, do not absorb moisture in humid environments. The materials could still arrive wet at an automated textile sorting facility if the textiles were warehoused outside, however.
Dry and wet samples of cotton and lyocell were inspected to test the effect of moisture on accurate material classification. The experiment determined that attempting to use the same classification algorithm for both wet and dry fabrics resulted in incorrect material identification. Very wet samples produced very noisy results. The researchers concluded that reliable classification for automated processing depended on dry textiles for analysis.
According to Prizztech advisor Essi Vanha-Viitakoski, the research study determined that most common recyclable textiles like cotton, wool, polyester, and blended materials based on these textiles were easily identified and sorted. The problematic materials cited in the study represented only a minor part of the full selection of test samples. The encouraging results provide further motivation to drive the development of these technologies, adds Herrala.
About the Author
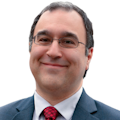
Dennis Scimeca
Dennis Scimeca is a veteran technology journalist with expertise in interactive entertainment and virtual reality. At Vision Systems Design, Dennis covered machine vision and image processing with an eye toward leading-edge technologies and practical applications for making a better world. Currently, he is the senior editor for technology at IndustryWeek, a partner publication to Vision Systems Design.