Sophisticated vision-based AI powers assistive device for the visually impaired
Persons with blindness or visual impairments face significant challenges when navigating everyday environments, and the most common assistive tools provide the user with limited information. A cane detects the presence of an object, not the object’s identity. A service dog can guide its owner away from danger, not specify the precise nature of the hazard.
An artificial intelligence (AI) based visual assistance system invented by Jagadish K. Mahendran, that won the OpenCV Spatial AI 2020 Competition sponsored by Intel (Santa Clara, CA, USA; www.intel.com) combines cameras, a suite of neural networks running multiple deep learning models, GPS, and voice interface to provide detailed and specific information about the locations of road signs, crosswalks, curbs, pedestrians, vehicles, and locations stored in memory.
Mahendran has since founded the MIRA Guidance for the Blind organization, currently raising funds and accepting volunteers toward development of the system.
It features two Luxonis (www.luxonis.com) OAK-D board-level camera units installed in a vest; a backpack that contains a Lenovo (Quarry Bay, Hong Kong, China; www.lenovo.com) Yoga laptop with an Intel i7 processor, 8 GB of RAM, and an Intel neural compute stick; a fanny pack that holds a battery that can run the system for eight hours; a USB-enabled GPS unit at the top of the backpack; and a wireless Bluetooth-enabled earphone.
Each OAK-D unit features an embedded Intel Movidius chip, one RGB camera, and two stereo cameras. The RGB camera has a 12 MPixel Sony IMX378 image sensor that can capture up to 60 fps, and a 1/2.3-in. lens. The stereo cameras each use an OmniVision OV9282 black-and-white CMOS 1 MPixel image sensor with 3 µm pixels that can capture up to 120 fps and a 1/2.3-in. lens.
The OAK-D acts as a smart camera and runs some of the system’s object detection and classification models. The Lenovo laptop runs the remaining object detection and classification models, the semantic segmentation models, and the GPS location system.
Mahendran used several open-source datasets and models. The OAK-D cameras include a pre-trained model for object detection using the Pascal Visual Object Classes dataset that can recognize up to 20 image classes including person, car, and dotted line. The SSD Mobilenet and TrafficSignNet models were used for object classification tasks.
For image segmentation, the system relies heavily on Advanced Driver Assistance Systems (ADAS) models, used in the development of autonomous vehicles, which are included with the OpenVINO toolkit. The semantic segmentation ADAS model includes vegetation, electric poles, and traffic lights and signs. The RoadSegmentation model can recognize sidewalks, curbs, roads, and road markings such as crosswalks.
In addition to the open-source datasets, Mahendran and his team took to the streets of Monrovia, California, to capture and label thousands of custom images for model training because the images were not available in open-source datasets or even via Google image searches.
“The existing models, the images are not taken from sidewalks,” says Mahendran. “Sometimes the dataset has to change according to the problem that you’re solving. The AI has to see the actual representation of the problem.”
Images of curbs, for instance, were high on the list of needed training images according to Breean Cox, Co-Founder of the MIRA Guidance for the Blind organization. Cox is blind and provides invaluable end-user perspective for the system’s development, says Mahendran, for instance the need to include curb detection algorithms.
“Curbs are very dangerous for blind people, falling off of them, tripping up them, it can lead to death in the worst cases,” she says.
“For sidewalks, for elevation changes, there are no standard datasets for curb detection,” says Mahendran. “I had to walk along the sidewalks and step up and down off curbs to collect the pictures along with the depth images. This is something we had to do from scratch.”
Stereo depth imaging determines the distance of all objects and obstacles from the cameras. The wearer determines the distance at which the system will announce its object detections using computer-generated voice and specific labels like “car” or “pedestrian.”
The deep learning models constantly run in parallel. For instance, when one of the object recognition models recognizes a Stop sign, the system announces “Stop sign.” This also triggers a semantic segmentation model to look for road markings like crosswalks. Upon detecting a crosswalk, the system announces “Enabling crosswalk.”
The system also announces the presence of random obstacles and their relative location, for example announcing “center” or “top front” if a wire or tree branch hangs in the camera’s FOV.
While front-facing cameras can detect the presence of a stop sign and a crosswalk, they cannot detect the presence of a car that is crossing against a light and about to enter the crosswalk from outside the cameras’ FOV. In this aspect, development of Mahendran’s visual assistance system could mirror the challenges faced by autonomous vehicle developers.
The system also features voice-activated commands. The wearer can ask for a description of their environment and hear a run-down of all the objects in the camera’s FOV via clock positions—“Pedestrian, 11 o’clock; road sign, two o’clock; car, three o’clock,” etc.
The wearer can also drop a GPS marker at a location using a voice command. When asked for nearby locations, the system announces those locations and their distances. While not the same as full GPS navigation, the location-saving feature provides orientation cues, another invaluable benefit according to Cox.
“I can be walking a block from my house and if something distracts me or gets me turned around, I can completely lose directionality and have no idea where I am,” says Cox. “If I am not paying attention, I can even forget which street I’m on. For a blind person, we have to always be mapping in our mind where we are. Even though [the system may only tell you] you’re 200 feet from a mapping point, to reorient yourself is very helpful. That also gives each person the ability to create their own maps and decide what’s important to them as they navigate around.”
Currently, among other challenges, the MIRA Guidance for the Blind team wants to make the system more comfortable and even less obvious to an observer. Unlike guide dogs or canes, the system does not easily draw attention to itself as an assistive device. The cameras rest inside the vest. Backpacks, fanny packs, and Bluetooth earpieces are common pieces of clothing and devices. In the hustle and bustle of a city, passers-by may not even hear the wearer issue voice commands.
In the end, the amount of control that Mahendran’s technology represents compared to these traditional methods is more important than transparency. “A guide dog is leading you through an obstacle course,” says Cox. “This is giving the decision making to the person’s hands, and they have to make the choices.”
About the Author
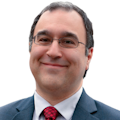
Dennis Scimeca
Dennis Scimeca is a veteran technology journalist with expertise in interactive entertainment and virtual reality. At Vision Systems Design, Dennis covered machine vision and image processing with an eye toward leading-edge technologies and practical applications for making a better world. Currently, he is the senior editor for technology at IndustryWeek, a partner publication to Vision Systems Design.