Deep learning system provides primary care physicians with melanoma screening tools
Fourteen researchers representing eight universities and organizations have collaborated on a new automated melanoma screening system, as described in the research paper, “Using deep learning for dermatologist-level detection of suspicious pigmented skin lesions from wide-field images (bit.ly/VSD-MNMA),” that addresses the shortcomings of current computer-aided diagnosis (CAD) tools.
Early-stage melanoma screening involves a methodology called outer lesion macroscreening, examining lesions for asymmetry, border unevenness, color distribution, diameter, and evolution (ABCDE) and how these factors change over the course of multiple examinations. These criteria support the “ugly duckling” screen method, examining a group of lesions to see if one of them looks different from the others. The lesion that looks different, the ugly duckling, is then assessed by ABCDE criteria to determine whether to biopsy or excise the lesion.
Primary care physicians (PCPs) form the first line of defense to make sure patients see dermatologists as early as possible after detecting suspicious pigmented lesions (SPLs). Vision systems and deep learning technology are well-suited for early-stage melanoma screening. However, current computer-aided diagnosis tools based on convolutional neural networks (CNNs) suffer from two key shortcomings.
First, these systems require preselection of specific lesions for the CAD system to analyze because the CNNs train on datasets of individual lesions. This assumes that a PCP has the training and time to determine all the appropriate lesions to select. Second, these systems also analyze individual lesions only, making the ugly duckling method impossible.
However, the new vision system developed by the researchers, optimized for analysis of wide-field images for rapid detection of SPLs and ranking by level of suspiciousness, can use the ugly duckling comparison method.
The training dataset of 33,980 images was gathered from open-access dermatology repositories, images scraped from the Web, and clinical images from 133 anonymous patients at the Hospital Gregorio Marañón (Madrid, Spain; www.comunidad.madrid/hospital).
The images were individually labeled into six object classes: backgrounds like furniture, walls, and other objects; skin edges; bare skin sections; two categories of nonsuspicious pigmented lesions (NSPLs); and SPLs. The NSPLs categories were lesions for which low-priority management is typically indicated and lesions that usually warrant follow-up exams or referral to a dermatologist. The SPLs were broken into categories by type and whether or not biopsy or excision are usually recommended.
Four deep convolutional neural networks (DCNNs), including one trained using transfer learning from the VGG16 ImageNet pretrained network that leveraged ImageNet’s 14-million image dataset and another based on the ImageNet Xception pretrained network, were trained and tested. A VGG16 transfer learning bottleneck model was chosen for the proof-of-concept demonstration.
One hundred thirty-five wide-field images depicting arms, full back, and full stomach, taken from 68 individuals, were used for the demonstration. Three board-certified dermatologists provided ground truth data for comparison, ranking legions for oddness. Dermatological consensus determined the average ranking of each lesion.
The system conducted its analysis in two fashions, according to Dr. Luis R. Soenksen, from the Massachusetts Institute of Technology (Cambridge, MA, USA; www.mit.edu), one of the researchers on the project.
“It evaluates every lesion ‘independently’ from other lesions to assess suspiciousness. In that task our system has 90.3% sensitivity [true positive predictions] and 89.9% specificity [true negative predictions] as compared to the consensus of dermatologists,” says Soenksen.
“It [also] evaluates every lesion in the context of all the other lesions in that same patient to calculate an ‘oddness score or ugly-duckling score.’ In that task our system achieves an 82.96% agreement with the same oddness scores produced by dermatologists,” continues Soenksen.
In the study’s conclusion, the researchers state that other avenues of system evaluation remain, such as the accuracy of the blob detection framework. It is expected to be robust, however, owing to its use of OpenCV libraries and the fact that every blob-like point extracted from the images by the DCNN was analyzed and confirmed as either an NSLP or SPL. This suggests a minimum number of unevaluated lesions in the images.
About the Author
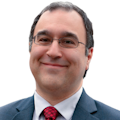
Dennis Scimeca
Dennis Scimeca is a veteran technology journalist with expertise in interactive entertainment and virtual reality. At Vision Systems Design, Dennis covered machine vision and image processing with an eye toward leading-edge technologies and practical applications for making a better world. Currently, he is the senior editor for technology at IndustryWeek, a partner publication to Vision Systems Design.