Researchers at the Visual Intelligence Laboratory in the Department of Mechanical Engineering at KAIST University (Daejeon, South Korea; www.kaist.ac.kr/kr) developed a new multi-view stereo method, called EOMVS, that uses omnidirectional event cameras to reconstruct 3D scenes with a wide FOV.
Event-based image sensors only register changes in pixel intensity. This unique nature of event-based cameras, according to the researchers, makes conventional computer vision algorithms for RGB frame-based images, including omnidirectional images, impossible to apply. Therefore, the researchers required a unique algorithm to process event-based images and enable their use for simultaneous localization and mapping (SLAM) or visual odometry (VO) applications.
To create a real-world dataset for use in their experiments, the researchers used a DVXplorer event camera from iniVation (Zurich, Switzerland; www.inivation.com) that features 640 × 480 resolution. An Entaniya (www.entaniya.co.jp) Fisheye M12 lens was attached to the event camera using a C-mount adapter, giving the camera a FOV up to 180°. The OptiTrack system developed by NaturalPoint (Corvallis, OR, USA; www.naturalpoint.com) obtained the pose information of the camera.
First, the researchers created a new event-based camera calibration technique using an accumulation method that defines the degree of contribution for each event pixel value and a decay function. The technique suppresses events otherwise likely translated as image noise.
Next, they created a method to allow depth estimation via event-based omnidirectional images. A disparity space image (DSI), a voxel grid, was constructed for each reference viewpoint (RV). This grid tracked projection rays of 2D events into 3D space from nearby viewpoints, as seen from the RV.
Voxels with the greatest intersections of rays were considered most likely to occupy a 3D point in the scene. Gaussian thresholding, which creates binary images from grayscale images, created a confidence map as to the location of those 3D points. The confidence map formed the basis of a semi-dense depth map and accompanying 3D point cloud that were overlaid on the original omnidirectional image.
The image processing took place on a PC featuring an Intel (Santa Clara, CA, USA; www.intel.com) Core i7-10700 @ 2.90 GHz 8-core CPU. Tests against synthetic indoor RGB and events datasets created using Blender 3D graphics software were followed by testing with real world images. An OSI-128 3D LiDAR unit from Ouster (San Francisco, CA, USA; www.ouster.com) obtained a ground-truth depth map for comparison against depth maps created by the EOMVS method.
Relative error fell between 4.7% and 9.6% as the FOV increased from 145° to 180°. The researchers now plan on developing a VO algorithm for the omnidirectional event camera to combine with the EOMVS method and thus enable omnidirectional event camera-based SLAM applications.
About the Author
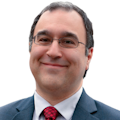
Dennis Scimeca
Dennis Scimeca is a veteran technology journalist with expertise in interactive entertainment and virtual reality. At Vision Systems Design, Dennis covered machine vision and image processing with an eye toward leading-edge technologies and practical applications for making a better world. Currently, he is the senior editor for technology at IndustryWeek, a partner publication to Vision Systems Design.