INVE Aquaculture (Dendermonde, Belgium) has launched SnappArt—a tool to automate the process of quantifying and assessing live feed, which is used to nourish farm-raised fish and shrimp.
SnappArt replaces conventional manual counting with a method that requires significantly less time and effort while delivering greater accuracy.
Aris BV (Eindhoven, the Netherlands), a machine vision systems integrator specializing in work for the horticulture and meat processing industries, developed the SnappArt System on behalf of INVE Aquaculture.
The Eurostars program, which provides funding for R&D projects that lead to innovative products, supported the development of SnappArt, which is now available commercially.
The Fish Farming Process
Live feed such as Artemia are hatched in saltwater to produce a certain larvae stage (nauplii), which are rich in nutrients, to feed a wide variety of juvenile fish and shrimp. Ideal fish and shrimp growth fundamentally depends on meticulous feed management.
The fish farming industry is growing due to increasing global demand for seafood combined with overfishing in wild populations, driving the need for alternative, sustainable sources of fish. Aquaculture offers a controlled environment that can significantly enhance fish production and reduce the industry’s impact on the environment.
Fish farming starts at hatcheries where larvae are hatched from fish eggs and are then nurtured in controlled environments until they are robust enough to survive in larger farming operations. The live feed is an essential nutrient to the larvae in these hatcheries.
Related: Spectral Imaging System Enables Digital Plant Phenotyping
Shortcomings of Manual Quality Control
Without an automated process to inspect and assess live feed, quality control of live feed such as Artemia and Rotifers relies on labor-intensive and error-prone procedures conducted on limited sample sizes. Typically, Artemia and Rotifers are counted manually under a microscope, a job that skilled staff can do in five minutes per sample.
Besides inconsistent feed administration, bad quality control can ultimately lead to poor growth and susceptibility to diseases for the fish and shrimp and economic losses for the marine hatcheries and farms, and negative environmental consequences for society. Moreover, skilled staff are hard to find, and counting methods are not globally standardized.
How the Vision-Enabled Automated System Works
At the heart of SnappArt lies its AI-driven image analysis software, ensuring rapid and precise analysis of samples. Coupled with an intuitive touch-screen user interface, the device facilitates seamless operation for hatchery personnel.
The initial steps in the automated process with SnappArt are like those used in manual counting methods. Operators first take a subsample from a hatching tank to estimate the density, stages, and quality of the feed. These are transferred to a microwell plate with 12 cells, which equals one sample. Each well has a diameter of 20 mm and contains as little as 350 uL sample volume.
Related: Hyperspectral Imaging Guages Salmon Maturation for Fish Farming
The SnappArt device is optimized on this well size. It uses a 12 MPixel camera, the MER2 series from Daheng Imaging (Beijing, China) with a Sony IMX226 sensor. Because of the high magnification, a C-mount macro lens from NEW TRY (Shenzhen, China) was chosen to obtain good optical performance of 6.6 um per pixel from the center to the edge of the well.
All the image processing is done locally on a NVIDIA (Santa Clara, CA, USA) Jetson Orin Nano module, which employs a YOLO object detection neural network as well as some traditional computer vison methods to recognize and count the live feed.
The individual components of the device are housed in a custom plastic casing designed to withstand the saline environment of hatcheries. Additionally, the carrier board from Forecr.io (Tallinn, Estonia) is treated with a conformal coating for protection against adverse conditions.
SnappArt can count a sample in just over a minute and then results are available immediately. They can be read from the screen, which might be enough for remote or small hatcheries, or the cloud.
For the cloud option, the counts of each separate stage and each well of the Artemia or Rotifers are sent to a Microsoft Azure database using a MQTT protocol over Wi-Fi. The data are then uploaded to a Microsoft Power BI application with customizable dashboards to analyze statistics about quality over time. The data is updated continuously.
As an added benefit of the system, hatcheries could use data collected over time to help measure overall productivity outcomes as well as any anomalies in the fish and shrimp.
Development of the Neural Networks
For the development of the deep learning object detection networks, data were collected from multiple locations worldwide and labelling was discussed with multiple experts. Interestingly, the identification of the different stages of live feed development was not standardized. This led INVE and Aris to develop uniform definitions of the stages, and multiple locations and experts agreed on these definitions.
On the other hand, the sample preparation method is now standardized, and the resulting data are well conditioned. For this reason, the neural networks were able to classify Artemia and Rotifers successfully with modest datasets of a few hundred images.
Related: The Rapid Rise of Computer Vision
For the annotation of images, CVAT, an open-source computer vision annotation tool, was used. Aris used an active learning approach in which predictions of models are used as a pre-annotation. This saved time because the models already had an accuracy above 90% early in the development process and only few annotations had to be added or corrected. The final accuracy is above 98%.
Also, INVE Aquaculture and Aris have kept a representative dataset, which they plan to use to validate performance after each update to the models. Validated models are updated over a cloud portal.
SnappArt signifies a transformative shift in live feed management, offering hatcheries a system that helps them enhance profitability, optimize growth ratios, and elevate overall production efficiency.
About the Author
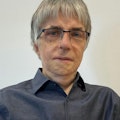
Rien den Boer
Rien den Boer is general manager of Aris B.V. (Eindhoven, the Netherlands), a company that provides computer vision solutions in the field of horticulture, aquaculture, and food. He started his career as an electronic engineer (Msc) in digital television at Philips NV and in 1996 became one of the founders of Aris BV. In 2019 Aris joined the Ellips Group (Eindhoven, the Netherlands). He is still involved in designing and architecting computer vision solutions.