Artificial intelligence software expands capabilities of Boston Dynamics’ Spot robot
AI Capabilities of Boston Dynamics Robot Dog Spot
Perhaps the most well-recognized robot of its kind, the Spot autonomous four-legged robot from Boston Dynamics (Waltham, MA, USA; www.bostondynamics.com) now offers on-board artificial intelligence software to process data and draw insights out of the environment while keeping human operators out of hazardous environments.
In September 2019, Boston Dynamics released Spot (Figure 1) to the world as its first commercial product to enable non-academic and non-military users to explore what this type of nimble, four-legged robot can do as a commercial application. While early adopters successfully deployed Spot robots for data collection, knowing how to understand that information and turn it into actionable insights quickly became a challenge.
“Spot can maneuver unknown, unstructured, or antagonistic environments and can collect various types of data, such as visible images, 3D laser scans, or thermal images,” says Michael Perry, Vice President of Business Development at Boston Dynamics. “Customers were thrilled with Spot’s ability to capture images of analog gauges, for example, but they soon expressed a need for the robot to relay that information to a work order system so that when a gauge reaches a certain level, it triggers a work order for an employee to follow up and inspect or perform maintenance.”
Related: What is deep learning and how do I deploy it in imaging?
Seeking to provide Spot with the ability to understand the data it collects; Boston Dynamics launched an early adopter program. When custom computer vision software company Vinsa (Palm Beach Gardens, FL, USA; www.vinsa.ai) applied, Boston Dynamics saw a strong fit for a partnership and the two companies began working together. Together, Boston Dynamics and Vinsa have deployed Spot equipped with Vinsa software on electric utility sites, oil and gas sites (Figure 2), and some chemical manufacturing partners as well, according to Perry.
Before the software can work, image acquisition must take place. Spot comes standard with five stereo cameras with global shutter, greyscale image sensors embedded into the body – two on the front, one on each side, and one on the back. Each of the five camera pairs feature a textured light projector and point at the ground to provide the robot information on where it can put its feet next to stay stable, while also providing obstacle avoidance capabilities. Spot perceives anything above 30 cm in height as an obstacle and avoids or walks around it.
Additionally, the robot uses these cameras for simultaneous localization and mapping (SLAM) for autonomous navigation. During mapping missions, Spot navigates through a space and creates a 3D point cloud of the space to get waypoints within the map. This helps the robot know where to go and what behaviors to perform. A stitched video of the five stereo cameras provides live video feed and a user interface provides access to all cameras.
Spot also has two DB25 ports that provide power and communications to external payloads. Any robot deployed with Vinsa software also features an onboard graphics processing unit (GPU) for inference, as well as a high-resolution, 360° pan-tilt-zoom camera which proves useful in situations where the robot must capture a high-quality, zoomed in image to allow the software to read a gauge, for example.
Related: Could vision-guided robots be key to keeping the restaurant industry afloat?
Vinsa’s main product line consists of pre-built deep learning models used primarily for industrial inspection use cases ranging from reading gauges (Figure 3) to detecting oil or water leaks. The company also has a patented engine called Alira which optimizes the performance of the computer vision models by including human operators via an alert system to help the models adapt to varying conditions. Both software offerings deploy into the Spot robot.
“Right now, the two companies are working with a customer that has approximately 100,000 analog gauges, which are very expensive to replace,” says Daniel Bruce, Founder and Chief AI Officer, Vinsa. “With this software, Spot can navigate to each gauge and capture images, and the software converts that into a digital reading using a built-in optical character recognition tool, so plant operators don’t have to do manual readings.”
In an environment with 100,000 gauges, all sorts of novel situations pop up. Glare on a gauge makes it hard to read, if a gauge is broken or bent, or if the robot encounters a gauge it hasn’t seen before, the software must decide what to do and how to adapt. Vinsa’s Alira engine handles situations like these by including a human subject matter expert and asking for help to handle such situations better in the future. Alerts depend on operator preference and come through the human-machine interface, through a text message alert, through e-mail, or through platforms like Amazon or Google, which have pre-built software for handling such situations.
Vinsa’s software leverages industry frameworks like YOLO (https://bit.ly/VSD-YOLO) or Inception (https://bit.ly/VSD-INCEP) which build on top of TensorFlow (www.tensorflow.org). The engine uses deep neural networks and over time as it sees more examples and instances, it improves by learning. Models train over a period of 30 to 45 days, where Vinsa calibrates its base intelligence layers around customer-specific environments by identifying the appearance of normal equipment to establish a baseline and decide when to raise an alert when something falls out of that baseline.
“It is important to mention that one thing Vinsa is not doing during this training period is trying to find all corner cases [situations that occur only outside of normal operating parameters],” says Bruce. “Many corner cases exist in production and some companies take a different approach by spending much more time training a model to learn all such cases.”
He continues, “What is unique about the Vinsa model is that it trains on these cases as they come up by looping in human expertise, which allows us to get models into production much faster, but also in a safe way where clients know when the models identify something previously unseen.”
Training consists of both images and video data, the latter of which gets synthesized into individual frames. For instance, detecting a leak in a pipe may not be possible by looking at a static frame. It may be necessary to see the sequence of a water droplet falling from a location in a pipe, something only detectable by video data, explains Bruce. Base models train on anywhere from 10,000 images to several million. During the calibration period, Vinsa generally looks for 2,000 to 3,000 examples of customer data to establish a baseline.
Processing can be done in three different modes, including edge processing, where all inference and models run on Spot on an onboard GPU. The system also supports on-premise processing, where Spot streams digital data back to a central location for processing, and specific communication gets pushed back to Spot. Lastly, the system supports cloud processing, such as on a virtual private network on Amazon Web Services (AWS; Seattle, WA, USA; www.aws.amazon.com), for example.
Not only does this collaborative effort provide additional benefits for customers in terms of productivity, it also takes human workers out of harm’s way, such as sending people to do screenings in a nuclear facility, doing rounds inside a containment zone, or dealing with highly-pressurized equipment on an oil rig. In the last example, notes Bruce, the company must depressurize the equipment and shut down operations to make it safe enough for humans to go in.
“Not only are there the soft savings of not putting people into dangerous scenarios, but also the hard savings of being able to inspect equipment that is in full operation without having to shut down. This results in higher uptime, productivity levels, and efficiency.”
About the Author
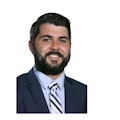
James Carroll
Former VSD Editor James Carroll joined the team 2013. Carroll covered machine vision and imaging from numerous angles, including application stories, industry news, market updates, and new products. In addition to writing and editing articles, Carroll managed the Innovators Awards program and webcasts.